Marketing Data Analytics Strategy Jan 3, 2018
Podcast: How Amazon’s Alexa Learns
Plus, an algorithm that can identify new social-media hashtags as they emerge.
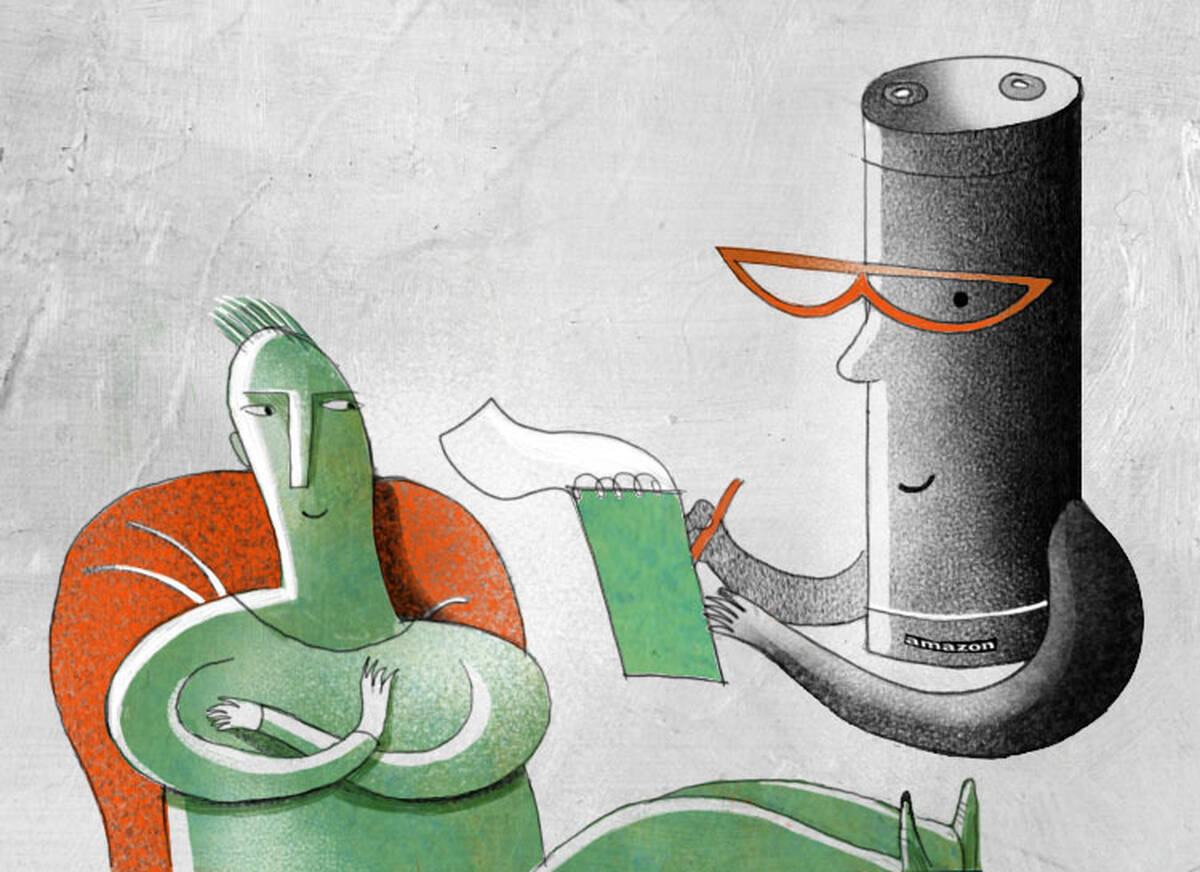
Yevgenia Nayberg
Much of the ballyhoo around intelligent home assistance devices is that they make life easier for us: from regulating our thermostats to freeing our hands while we check a favorite recipe for roast turkey, to playing that favorite jam to get us pumped up in the morning. And it turns out that because these devices are designed to learn from our patterns and habits, they become more helpful the longer we live with them.
In this episode of the Kellogg Insight podcast, Ashwin Ram, one of the minds behind Amazon Alexa, describes how the company is balancing privacy concerns with natural language recognition to design a more effective device.
Then Jennifer Cutler, an associate professor of marketing at the Kellogg School, describes how she and her colleagues are making large-scale analysis of social media trends a lot faster and easier.
PODCAST TRANSCRIPT
Jessica LOVE: We’re all familiar with the learning curve that accompanies any new gadget we buy—whether it’s a smartphone or an espresso maker. No matter how user-friendly the product is, we know that it’s going to take some time—and probably some frustration—to figure out what all of the buttons do.
And we just kind of accept that fact.
But Ashwin Ram doesn’t.
Ashwin RAM: I kept thinking, when we talk to each other, we don’t do that. We just talk. Why can’t I just talk to these machines and have them do the right thing for me? Why do I have to learn to talk to them? Why not have them learn to talk to me?
LOVE: Ram is an artificial intelligence expert. He’s one of the minds behind Alexa, the voice-activated personal assistant from Amazon that can do everything from controlling the lamp in your living room to ordering a pizza.
As Ram points out, you don’t have to learn much about how Alexa’s algorithm works in order for it to be useful.
And what learning curve does exist is designed to be ... fun.
RAM: People report that Alexa feels like a friend or a family member almost. People like interacting with Alexa, and there’s actually a personality team that has designed the personality of Alexa and maintains that. So Alexa has a particular way of speaking, particular quirky sense of humor. There’s a whole backstory behind her character, and you can uncover that if you ask a little bit about Alexa and so forth.
LOVE: That’s right—if you are interested in learning more about Alexa, you can just ask Alexa, using regular, conversational language.
And one of the things that makes Alexa so innovative is the fact that it is also using regular, conversational language to learn about us.
Welcome to the Kellogg Insight podcast. I’m your host, Jessica Love. In this episode, we’re going to speak with Ashwin Ram to learn more about how Alexa learns.
We’re also going to hear from Kellogg professor Jennifer Cutler. She’ll discuss a technique she’s developed that allows algorithms to glean key information about us from our conversations on social media.
So stay with us.
[Music interlude]
RAM: Our goal is that you should not have to change the way you talk to be able to interact with Alexa.
LOVE: That’s Ashwin Ram again. Here’s an example of what he means. Let’s say you can’t remember the details of your favorite baseball team’s performance last season. You can just blurt out, “Alexa, what was the Cubs’ record this year?”
ALEXA: In the 2017 season the Cubs finished first in the National League Central with a record of 92 and 70. The Cubs won four playoff games this year. They were eliminated in the National League Championship Series.
LOVE: Unfortunately for those of us in Chicago, Alexa can’t actually do anything to get the Cubs to the World Series again. But the abilities it showcases in that interaction are pretty impressive anyway, starting with how it homes in on your words.
RAM: Imagine you have maybe a bunch of friends over and you’re all talking and there’s music playing and dishes clanking and all of that, and someone says, “Alexa, what’s the weather like.” You have to now pick up that voice in that crowded environment and respond to that request accurately.
LOVE: And Alexa doesn’t just have to identify those words. It also has to figure out which meaning of those words you intend, so that when you say “Cubs,” it doesn’t start talking to you about baby bears instead of baseball players.
What makes Alexa’s job a bit less daunting is that there are some limits to the topics you’re likely to converse with it about.
RAM: It doesn’t have to interpret your opinions of Shakespearean plays. It’s focused on the kinds of things that you’re going to interact with this device about.
LOVE: Another thing that makes Alexa’s job easier is … us. By talking to it the way we usually talk, we’re teaching it, helping it become more capable of handling our requests correctly.
RAM: Every time you interact with Alexa, you are teaching Alexa something that helps Alexa work better with you but also with everybody else. There’s only one Alexa in the cloud. If my Alexa gets better, yours is automatically better.
If lots of people start talking to Alexa in a particular way, all of that will start filtering in in the models, and Alexa will get better at understanding that way of requesting something.
LOVE: Alexa’s also constantly learning thanks to the fact that anyone can give it new capabilities, what Amazon calls “skills.” Right now, third-party developers have added about 25,000 skills to Alexa, and that number grows all the time.
For example, its Uber skill lets you order a ride. Its Capital One skill lets you check your credit-card balance. And with its Pizza Hut skill, you can order dinner.
By the way, you don’t have to be Capital One or Pizza Hut in order to give Alexa new capabilities. Amazon taught Alexa to speak American English, British English, and German. But with a skill called Cleo, you, the user, can help it learn additional languages—Russian, Bengali, whatever you want.
ALEXA: Welcome back! Would you like to continue teaching me French?
LOVE: It’s not clear yet how successful this crowdsourcing project will be. From Amazon’s point of view, of course, it would be great to have users teach Alexa to parler français, so it can launch in France with a lot less work on Amazon’s part. Time will tell.
[Music interlude]
So a lot of what Alexa is learning benefits all Alexa users. But there are some things it learns about you in particular. Because even though there is only one Alexa in the cloud, it does track some information about individual users. Like where you live—helpful if you want to know tomorrow’s temperature. Or what requests you and your family members have made in the past.
RAM: As a very simple example, if you’re listening to some music on Alexa and you say, “Hey, that’s a nice piece of music. Add it to my music library.” You now have that music in your music library. The chances are pretty high that you will now ask for that piece of music or that band or that artist again.
LOVE: Let’s say you’re a big fan of Little Richard. There are lots of musicians named Richard out there, from Richard Marx to Richard Wagner. But because you specifically asked Alexa to add this piece by Little Richard to your library, the next time you ask for just plain Richard, it knows to pull up the right one.
This is the part where some consumers get nervous. Specifically, they’re concerned that Alexa might be learning things a user might not want it to know. It’s one thing if it knows that you’d rather listen to “Good Golly Miss Molly” than “Right Here Waiting.” It’s another if it’s retaining information from your private conversations.
Ram says this particular privacy concern is misplaced.
RAM: The most common misconception I hear—and I almost always asked about this when I give talks on Alexa—is this idea that Alexa is somehow eavesdropping on you all the time and it knows everything that’s going on. There are people who will put little sticky tape over the camera on their laptops because they think their laptops are spying on them through their cameras. This is a serious concern. This device is there, listens to voices, perhaps it’s listening to you.
LOVE: For the record, Ram says, Alexa’s microphone is not recording until it is triggered by the word “Alexa.”
RAM: Alexa does not listen to anything until you speak to Alexa. We are not streaming your voice up to Amazon to analyze it, to look for whether you’re saying Alexa or not.
LOVE: Ram stresses that Alexa doesn’t listen to anything going on in the house unless you are directly talking to it.
That fact won’t change, he says. But Alexa will continue to evolve.
RAM: The technology’s changing dramatically fast right now. It’ll be different in an obvious way and a not so obvious way. The obvious way is some of the techniques you use, for example, some of the deep neural network modeling techniques and speech-recognition techniques, etc. Those are constantly being adopted, adapted, evolved, experimented with. There’ll be better and better techniques from Amazon and from the academic literature as well that we will incorporate as things improve. The actual algorithms and the types of models you use in the Alexa of 10 years from now are likely to be different from what’s in there literally right now.
The other way that I think Alexa will be different is Alexa’s always learning. Always learning from your interactions and from all of the interactions. Alexa will learn and change over time just like anyone else learns and changes over time. It’s essentially a two-and-a-half-year-old child right now, and it’ll learn about its world and its users and slowly get better over time as well.
[Music interlude]
LOVE: Of course, as humans our conversations touch on a lot more than pizza orders and song requests. And they certainly don’t all happen aloud. So now we turn to another place where we like to communicate: social media.
For this segment, we go to Fred Schmalz.
Fred SCHMALZ: It can be relatively straightforward to look at an individual Tweet and tell what that particular user believes about a given topic.
But what if you want to parse a thousand Tweets, or a million? What if you want to parse a trend across the entire social platform—and on Instagram and Facebook too?
For marketers, having this kind of information on hand could be incredibly valuable. Here’s Jennifer Cutler.
Jennifer CUTLER: Maybe I want to predict what the personality of what my brand followers are. Maybe I want to predict the demographics of people who are interested in a certain topic on Twitter and who keep tweeting about #BIGDATA or something like that. Or maybe I want to track or predict the topic of different posts on social media and track conversations over time and figure out what platforms are having what conversations, and who are these people joining in on them.
SCHMALZ: Cutler, an assistant professor of marketing at the Kellogg School, is working to simplify and speed up the way that marketers mine consumer insights from social media.
Here’s a traditional method of getting insights from, say, Facebook or Twitter. First, you need something called “labeled data”—data that is essentially tagged with all of the information that a machine needs in order to make sense of it.
Obtaining labeled data is a big, expensive investment. Picture an army of research assistants combing through half a million tweets and marking which topics the tweets discuss.
With that mountain of information, marketers can start to make connections between the words people use and the topics they are interested in—or whatever other information has been labeled. They then can build a model to predict interesting things about consumers or tweets for which this information has not been labeled.
There’s just one problem.
CUTLER: The problem is that especially in social media, a lot of things change very rapidly.
SCHMALZ: Let’s say you’re a marketer trying to identify consumers’ level of interest in environmental sustainability. According to the model you made based on your labeled data, you should be tracking social-media users engaging with the hashtag #ECOFRIDAYFORTHEWIN. But in the time since you made that model, everyone abandoned that hashtag and started using #SUSTAINABLESATURDAY instead.
Darn.
According to Cutler, changing hashtags are a big problem, because hashtags do a lot of heavy lifting.
CUTLER: The reason why these become so important for things like text analysis, or understanding user perceptions, or understanding consumer behavior is that because a lot of social-media posts are so short, these very rapidly changing and evolving user-generated hashtags or other bits of slang are often the only indicator in a tweet about what the content is about. They might give an opinion like, “I love days like this #EARTHDAY.” And really, the hashtag is the only thing that’s giving you context about what they love.
SCHMALZ: But it’s really hard for this traditional model to work in the real world, where language is constantly evolving.
So Cutler has built a work-around—one that allows marketers or researchers to bypass the slow, hard work of acquiring labeled data.
Here’s the logic:
Rather than focusing on individual posts and whether they are about a given topic, focus on social-media accounts that are dedicated to that topic.
So if you’re trying to identify markers that signal someone has interest in the environment, you look at accounts like Greenpeace or World Wildlife Fund. What are they Tweeting about?
CUTLER: If we can get a whole bunch of accounts that we know will be speaking about the environment at a much higher rate than average, we can then compare the language they use against a random pool or a well-defined control set of similar accounts, but that aren’t known for their environmental friendliness.
SCHMALZ: Through that comparison, you can tell which hashtags or words or messages signal interest in the environment right now—as opposed to the ones that might have signaled that six months ago.
In fact, you can automatically capture new hashtags as they emerge.
The great thing about this approach is that not only does it yield the most up-to-date information, but it’s also a heck of a lot easier than the old way.
CUTLER: I have methods that I’ve developed with my computer-science collaborator where you just need to put in one key word. Just put in the word “sustainability.”
And it will go and find accounts that are organized by users into lists about sustainability.
And so when you have fairly popular topics like sustainability, there’s going to be hundreds, if not thousands, of lists that users have organically created to say, “Hey! I, the user here have deemed these accounts to be relevant to the environment. And I have curated this newsfeed.”
And so all we really have to do is actually mine these lists. And then the lists give us a very large number of accounts. And from those accounts, we can then get a large number of these proportionately labeled tweets. The beauty of this, compared to a lot of prior work in data mining, is that it’s fully automated and you can get a perfectly up-to-date model—the cleanest, most recent language—with just a single keyword of input.
SCHMALZ: There are endless ways marketers or researchers could use this information. For example, Cutler is using it to compare how environmentally friendly a brand actually is with how “green” they are marketing themselves to be on social media. She hopes this will help her better understand the nature of “greenwashing,” a term that describes intentionally creating a false appearance of being eco-friendly.
This new approach can also help marketers to determine which platforms are the most popular for certain topics of conversation. That way, you don’t waste effort on a Facebook campaign if the discussions most relevant to your brand are all on Instagram instead.
CUTLER: Something that I think is really exciting is that one of the implications of making data mining so scalable and flexible, and easy to apply for a wide range of tasks, is that we open the door to doing a lot of research about how consumers behave and how they interact with brands at a scale that’s really unprecedented.
SCHMALZ: While there are currently commercial tools that track conversations and influencers, none of them really reveal how they’re coming up with their results. In contrast, Cutler is very open about the algorithms she uses.
CUTLER: These are algorithms that we’re publishing in journals, and so they’re pretty transparent and shockingly not very complicated. The real innovation here is just instead of trying to impose all of this very-difficult-to-obtain training data and build a model, we’re just finding sources that users are already providing. And the whole point of what we’re doing is trying to make it really easy and transparent. So I don’t have a tool that I’m selling right now. All of this, for anyone who has some programming background, would be very, very easy to both replicate and tailor towards their purpose by reading the papers themselves.
SCHMALZ: If this approach is so useful and so accessible, why haven’t more marketers adopted it already? Cutler says it’s because many valuable insights from data science just aren’t migrating into the marketing world very well, either to practitioners or to academic researchers of marketing.
CUTLER: One of the bottlenecks has been that a lot of people working on data mining who are trying to extract insights from social-media data may not be aware of the resource constraints of everyday managers applying these tools.
And because, I think, the fields have been very separate—certainly the fields of marketing and data science. They’re not entirely separate. There are definitely areas of overlap, but I think that there really hasn’t been enough integration and communication between the two to really motivate this need for ongoing, real-time predictions.
SCHMALZ: And that’s a shame, she says, because this approach to learning about consumers yields incredibly useful insights.
CUTLER: Same thing with persuasion—we can see brands that are really pushing a message and it’s sticking and brands that are really pushing a message and it’s not sticking. And while it’s, there are a lot of factors that go into brand perception, so if you only compare two brands, you’re not going to get anything particularly generalizable. But because of the scale of this, we could look at hundreds of brands. And then patterns start to emerge.
[Music Interlude]
LOVE: This program was produced by Jessica Love, Fred Schmalz, Emily Stone, and Michael Spikes. It was written by Anne Ford.
Special thanks to our guests, Ashwin Ram and Jennifer Cutler.
You can stream or download our monthly podcast from iTunes, Google Play, or our website, where you can read more about advances in machine learning. Visit us at insight.kellogg.northwestern.edu. We’ll be back next month with another episode of the Kellogg Insight podcast.
Editor’s note: The audio version of this podcast episode characterizes an approach of using labeled data to mine social media for consumer insights as the only traditional method. However, it is just one of multiple traditional methods.