Data Analytics Organizations Aug 2, 2021
Gig Workers Are Increasingly Rated by Opaque Algorithms. It’s Making Them Paranoid.
These systems can create an “invisible cage” for freelancers.
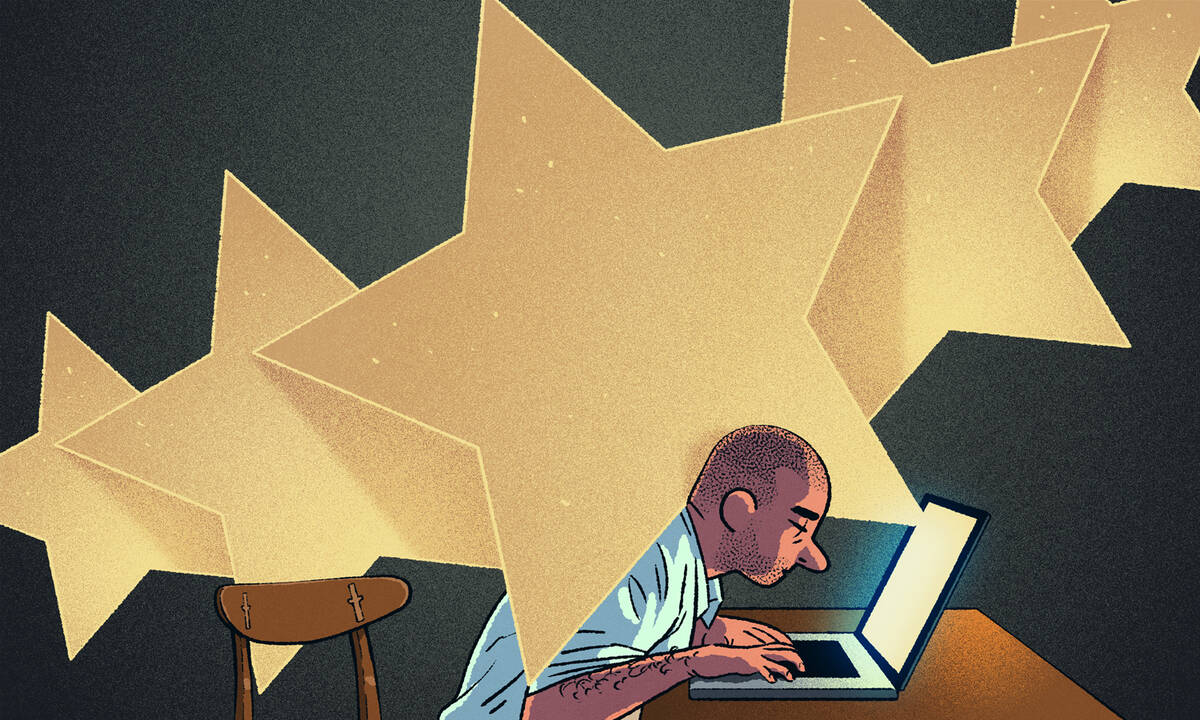
Michael Meier
In some corner of the Internet, you’ve likely already been rated.
These ratings help all kinds of businesses make decisions, like where to take risks and how to improve their operations. But how are ratings affecting the people who are being rated? New research from Kellogg’s Hatim Rahman suggests that despite these algorithms’ opacity—indeed largely because
of that opacity—they’re shaping people’s behavior in unexpected ways.
Rahman, an assistant professor of management and organizations, investigated the impact of algorithms in an online labor platform for freelancers. “There’s a lot of conversation about the future of work and what it will look like,” Rahman says. “These platforms are part of the next frontier.”
Like many sites that promise to connect freelance workers to paying clients (among them Upwork, TopCoder, and TaskRabbit), the one bearing Rahman’s scrutiny employed a complex algorithm to score freelancers. Potential clients could sort and select potential hires based on that score.
To find out how this opaque evaluation system was affecting the freelancers, Rahman joined the platform (which he refers to pseudonymously as “TalentFinder”) and conducted interviews with freelancers and the clients hiring them. He also parsed written communications from TalentFinder and posts on its freelancer discussion boards.
All of the workers he spoke with experienced ongoing paranoia about the possibility of a sudden and unaccountable score decrease. The way they responded to this fear was less dependent on the strength of their rating than on whether they’d previously experienced decreases in their scores—and, crucially, how dependent on the platform they were for income.
Traditionally, Rahman explains, scholars have described workplace evaluations as helping to tighten an “iron cage” around workers, because they allow employers to constrain behavior and set the standards of success. Algorithmic evaluations have a different, potentially undermining impact.
“Opaque third-party evaluations can create an ‘invisible cage’ for workers,” Rahman writes, “because they experience such evaluations as a form of control and yet cannot decipher or learn from the criteria for success.”
Of course, as Rahman points out, not only are many of us living in some version of this invisible cage—we also play a small part in shaping its bars. Every time we rate an Amazon purchase or a Lyft driver, we are potentially affecting others’ livelihoods.
“People using these platforms are largely unaware of the role they have in influencing these systems and its algorithms,” he says. “It just feels like a very transactional relationship.”
Making Evaluation Criteria a Mystery
“TalentFinder” is one of the largest platforms of its kind. In 2015, over 12 million freelancers were registered on the site, alongside 5 million clients based in over 100 countries. Clients could select from a wide range of gig workers, from assistants to marketers to software engineers.
When Rahman registered with TalentFinder to start his research in 2013, the platform rated freelancers according to a transparent system of project scores and overall scores. Upon completion of a project, clients would rate freelancers on a scale of 1 to 5 on a number of attributes, including “Skills,” “Quality of Work,” and “Adherence to Schedule.” Aggregating these scores resulted in an overall project score, and combining those project scores (weighted based on the dollar value of each project) resulted in an overall rating out of five stars, which was included in a freelancer’s profile.
As straightforward as this evaluation system was, it presented a problem for TalentFinder: freelancers’ ratings were too high across the board and lacked the differentiation that would make them helpful to clients. At one point, more than 90 percent of freelancers had at least four out of five stars, and 80 percent had a near-perfect rating.
“I think that the invisible-cage metaphor applies more broadly as we are entering into this system in which all of what we do, say, and how we interact is feeding into algorithms that we’re not necessarily aware of.”
— Hatim Rahman
The solution: an algorithm. Starting in 2015, freelancers were rated on a scale of 1 to 100, based on intentionally mysterious criteria.
“We don’t reveal the exact calculation for your score,” TalentFinder wrote in a public blog post three months after the algorithm’s introduction. “Doing so would make it easier for some users to artificially boost their scores.” After the implementation of the new algorithm, only about 5 percent of freelancers received a score of 90 or above.
To study the effects of the new evaluation system on freelancers, Rahman collected data between 2015 and 2018 from three sources: 80 interviews conducted with freelancers and 18 with clients; written communications, including over two thousand TalentFinder community discussion-board messages related to the algorithm and all of TalentFinder’s public posts on the topic; and his own observations as a registered client.
Pervasive Paranoia
As Rahman culled through his interviews and written sources, he was struck by the consistency of the complaints he heard. All of the freelancers he spoke with experienced paranoia around possible sudden drops in their scores and ongoing frustration over their inability to learn from and improve based on score fluctuations.
“What surprised me the most was that the highest performers and most experienced freelancers on the platform didn’t necessarily gain any advantage in terms of figuring out how the algorithm worked,” he says. “Generally, those who do well in a system are able to figure out what’s going on to some extent. But in this context, even people whose scores hadn’t changed were very much on edge.”
Rahman observed two distinct reactions to this paranoia and frustration. One reaction is what he terms “experimental reactivity”—freelancers trying through trial and error to increase their scores, for example, by taking on only projects with short contract lengths or by proactively asking clients to send feedback.
The other reaction was when freelancers attempted to protect their scores through what Rahman calls “constrained activity.” Freelancers tried through various means to limit their exposure to the evaluation algorithm, sometimes asking clients they met on TalentFinder to move off the platform to communicate and make payments so that their ratings wouldn’t be affected. Others did nothing in hopes that this would preserve their rating.
Rahman isolated two main factors that determined which freelancers experimented, and which pulled back from the platform or simply did nothing: the extent of a freelancer’s dependence on the platform for income, and whether they’d experienced a decrease in their score.
This varied by whether a freelancer had a high or low score.
High-rated freelancers with high dependence on the platform chose their tactic based on whether they had seen a recent drop in their score. Those who had seen their score drop experimented with tactics to raise it; if their score had not dropped, they constrained their activity on the platform in an effort to protect their score. High performers with low dependence on the platform constrained their time on TalentFinder, whether or not they’d experienced a score drop.
For freelancers with lower scores, their dependence on the platform appeared to determine which path they took. If they depended on the platform, they engaged in experimentation even if scores continued to fluctuate. If they didn’t feel as tied to it for income, they gradually constrained their activity.
Rahman explains that the workers’ position feels more precarious on these platforms than in traditional work settings because, well, it is. While typical employer evaluations are largely meant to help an employee improve, the evaluations facilitated by an algorithm on a site like TalentFinder are primarily meant to help the platform automate the job of plucking the “best” workers from an enormous pool, thereby satisfying its clients.
“For the platforms, it’s about them optimizing their overall dynamics; their primary goal is not to help workers improve,” Rahman says. “For people’s day-to-day lived experiences, especially when they’re relying on the platform for work, this can be very frustrating and difficult.”
Living in the Cage—and Shaping It
Since conducting this research, Rahman says he’s increasingly clued into the various invisible cages within which most of us live. He points, for example, to recent reports detailing how everything from our TVs and vacuum cleaners to the smartphone apps we use for medical prescriptions
and car insurance are collecting our data and using it to train proprietary algorithms in ways that are largely invisible to us.
“I think that the invisible-cage metaphor applies more broadly as we are entering into this system in which all of what we do, say, and how we interact is feeding into algorithms that we’re not necessarily aware of,” he says.
He points out that some people are freer to withdraw from these platforms than others; it all comes down to their level of dependence on them. A fruitful area for future research, he says, could be in examining how characteristics like race, gender, and income correlate with dependence on platforms and their algorithmic evaluations. For instance, it’s important to understand whether individuals from certain racial groups are more likely to be “evaluated” (and potentially blacklisted) by algorithms before they can rent an apartment, open a credit card, or enroll in health insurance.
“The hope of bringing this invisible-cage metaphor to the forefront is to bring awareness to this phenomenon, and hopefully in a way that people can relate to,” Rahman says. “Of course, even when we become aware of it, it’s difficult to know what to do, given the complexity of these systems and the rate at which their algorithms change.”
Legislation is starting to provide some oversight in what remains a largely unregulated area. The 2020 California Consumer Privacy Act, the strongest such legislation in the nation, establishes online users’ rights to know about, delete, and opt out of personal data collection. In 2018, the European Union passed even more aggressive legislation to the same end. “It’s a heartening sign,” Rahman says, “but regulation alone is far from a panacea.”
Katie Gilbert is a freelance writer in Philadelphia.
Rahman, Hatim. 2021. “The Invisible Cage: Workers’ Reactivity to Opaque Algorithmic Evaluations.” Administrative Science Quarterly.