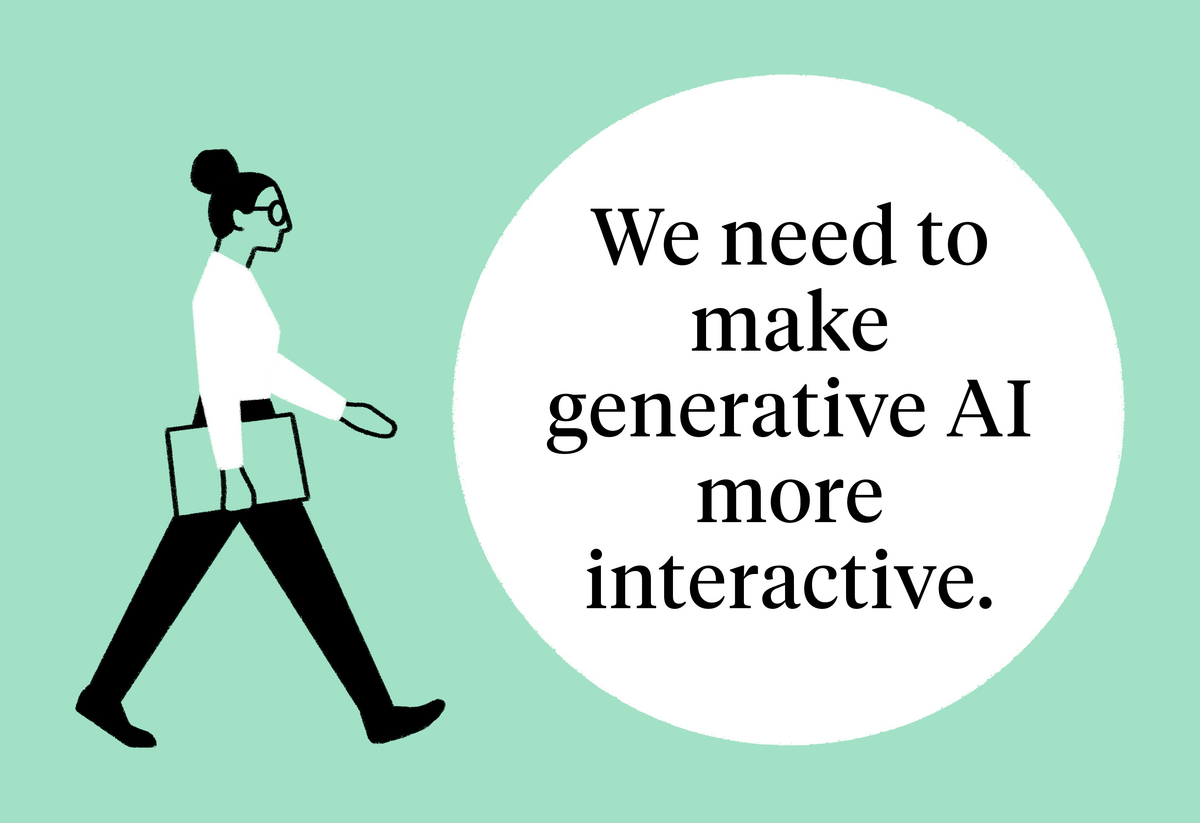
With the right combination of prompts, generative AI can create animated films and (possibly) even write the next great American novel, doing it all in a fraction of the time it would take humans, alone.
But the efficiency we gain from these tools can come at the cost of originality, says Sebastien Martin, an assistant professor of operations at Kellogg.
“At the population level, all of this guesswork that we leave to the AI will lead to something that’s a little bit more homogenized,” Martin says.
On this episode of The Insightful Leader: are we in for a world of sameness?
Podcast Transcript
Laura PAVIN: You’re listening to The Insightful Leader. I’m Laura Pavin. A year after ChatGPT busted into the mainstream, generative AI is still blowing our minds. It can write college essays and it can code, and it can create paintings and music.
It’s incredible stuff … and sort of scary too. So it is a subject that our team here at Kellogg Insight can’t stop talking about. Including our editor in chief, Jess Love, who just happens to be with me in the studio. Hi, Jess.
Jess LOVE: Hey, hey, great to see you, Laura.
PAVIN: So Jess, you messaged me a couple of weeks ago and said you had an AI story you wanted to talk to me about. I’ve been patiently waiting since then to hear about it—so what’s up?
LOVE: Yeah, I’ve been excited to finally get on the mics and talk about this one. So, it has to do with generative AI. And there’s been a lot of discussion about where this technology is headed, and how quickly it will continue to improve. But one question I’ve had for a while is, what happens if everyone starts using it? Like, if the majority of people around the world start using ChatGPT to write, or Midjourney to illustrate, what will this change about the nature of what we collectively produce?
PAVIN: That sounds like a pretty big question. How would you even start answering that?
LOVE: Well, we’re very fortunate that at Kellogg there is a professor who was wondering the same thing. His name is Sebastien Martin, and he got interested in this in part because he uses a program called Grammarly when he’s writing in English, which is his second language.
Sebastien MARTIN: Because it’s much, much faster for me to write correct English sentences that sound a little bit better compared to if I had to read it and read it again. So in terms of time, I save a lot of time.
PAVIN: Oh yeah, I know that program. That uses AI?
LOVE: Yup, it basically reads whatever you’re writing in real time and makes suggestions along the way.
PAVIN: Gotcha, so he uses this AI-powered tool and it saves him time…
LOVE: Right, but what he’s noticed is that what Grammarly might write or suggest isn’t very … personalized. To him.
MARTIN: What Grammarly will write will not be exactly what I would have written even given enough time. You know, punctuations, there are different ways to write the same thing. Sometimes it’s just a matter of taste. It’s idiosyncrasies.
PAVIN: Got it. What we in the journalism biz might call “voice.”
LOVE: Right, but with more powerful AI tools like ChatGPT, this can go beyond “voice.” It can capture important nuances about what we believe. So whether we describe an event as “protest” or a “riot,” or write a news article from a center-right versus a center-left slant, that captures something unique about how we personally see the world.
LOVE: And the thing about generative AI is, when you use it to help you produce content more quickly, you lose some of that uniqueness, which Sebastien refers to as fidelity: basically, how faithfully that output reproduces our own style or perspective.
PAVIN: Okay, that makes sense, and I think I see where he might be going with this. But continue.
LOVE: So. As more and more people start to use generative AI to create things, this means more people trading “fidelity” for productivity. And Martin wanted to know what this would mean at a societal scale.
PAVIN: And how does he plan to study that?
MARTIN: When you want to study a complicated phenomenon like this, especially at the scale of society, you cannot just rely on experimentation and asking a few people. You have to abstract away from all of this.
LOVE: Essentially, he and his colleagues built a mathematical model to simulate the consequences of an entire society using the same AI tools.
MARTIN: The reason why we choose a model, it’s because once we’re able to agree that this idea that we described can be relatively accurately represented by this model, then we can ask the model about a lot of things. Like what would happen in this case, in that case. What are the cases where potentially the introduction of AI is most dangerous?
LOVE: So Martin builds this model, where users choose how much they want to personalize the output. So on the one hand, you can just run with what you get, and accept it will be generic. Or you can choose to personalize it, but that takes a lot of work.
MARTIN: Either I rewrite stuff or I communicate more with the AI. And this has a cost: we call it the communication costs.
LOVE: And the model reveals a few things. For users with the most-common perspectives—maybe kind of a generic style anyway—the optimal thing is just to accept the AI output as is. Great! You’re done.
But for users with more-unusual perspectives—maybe a bit more pizazz—the optimal decision is to use the AI but … personalize it a lot.
PAVIN: Right, so these are the users who would tell the AI more information about exactly what they want, or maybe edit the output.
LOVE: Exactly. And then there’s a third group whose preferences are so unique that it probably doesn’t make sense to use the AI tool at all.
PAVIN: So they are better off just writing that paragraph or drawing that picture themselves.
LOVE: That’s right.
PAVIN: Fascinating. But so far we’re still talking about individual users, right? What happens when everybody starts using this thing?
LOVE: Well, the model simulated that, too. The biggest finding is: homogenization. When everyone collectively trades fidelity for efficiency—when we pull away from something that sounds closer to us and instead accept something that is “close enough”—things get a little … same-y.
MARTIN: And so this phenomenon overall leads to something we call homogenization. So you can see that at the population level, all of this guesswork that we leave to the AI will lead to something that’s a little bit more homogenized. All the situations for which we don’t give information, the AI will tend to return a little bit of the same thing.
PAVIN: Okay, so the AI will produce more-generic content than if the users had produced it themselves. The content is all just kind of … samesies.
LOVE: Yes, and this effect gets amplified because remember: all those picky, unusual people who want to edit the output so much that they might as well just do it all themselves, they’re less likely to use the tool at all. That makes the overall output even more generic.
PAVIN: Alright. So, should we be worried?
LOVE: Well, in addition to this output being more boring, we are also collectively losing a lot of important human perspectives and nuance. And here’s the thing: over time, it just gets worse and worse.
MARTIN: The next generation of AI, their training data will be this data, all these things that have already been generated by AI. But we just said that AI will generate things that are a little bit more homogeneous. So the next generation of AI will think the world is more homogenous than what it really is and will tend to even more push us towards something that’s more standardized.
PAVIN: Oh right, so like if the next generation of ChatGPT were trained on an internet that’s already full of writing generated by … ChatGPT.
LOVE: You nailed it. Researchers refer to this as a “death spiral,” where the negative effect compounds itself.
PAVIN: Death spiral sounds pretty bad!
LOVE: It’s definitely not good.
PAVIN: Okay, death spiral, adding it to my list of top fears. Anything else I should worry about?
LOVE: Yeah, so setting aside the issue of homogenization, something important for us to remember is that regardless of how amazing these tools are, at the end of the day they’re still made by humans. And a pretty small number of humans at that.
MARTIN: The truth is, a limited number of human beings have had a huge importance in the training of the AI. That’s true. And then, the AI is used by tens of millions or hundreds of millions of people to do whatever. So you have a small group of people that make a lot of decisions just by saying, this is good, this is bad, about how the AI will then react and output things and interact with people.
PAVIN: So because these programmers are training the AI to give one response over another or to not even engage with certain topics or prompts, we could see those choices reflected in more and more writing?
LOVE: Right, so it’s different but related to the concern about AI giving generic responses. Essentially, it’s another way in which writing could become less … representative.
MARTIN: Whatever little preferences, little biases, that this limited group of people had, it can then be broadcasted at the scale of the population. So any AI bias can turn into a societal bias.
PAVIN: Ah, so if the people training the AI are one political stripe or another, something like that might seep into our collective brains.
LOVE: Right.
PAVIN: So are there any solutions here? Do we just need to make the AIs smarter?
LOVE: Improving the technology could be one possibility, but Martin thinks there are ways forward by just changing how we interact with and design AI now. And a lot of that comes down to making these tools more interactive.
PAVIN: What do you mean?
LOVE: Well, the way we’ve been talking about using AI so far has been simple input and output. But you could also imagine AI asking users a few questions before generating a result, to learn more about their perspectives and preferences. Or always providing multiple options and asking you to choose one of them. Or maybe the AI could help users create their own output. Martin gave the example of a homework assignment.
MARTIN: There are two ways of using ChatGPT for your homework. You can either give the question and ask for the response; then you didn’t learn anything. Or you can ask ChatGPT to act as a tutor, like you were saying to ... help me write my homework. And then they will give you hints and guide you ... but at least there is an exchange and the output will be closer to who the student is.
PAVIN: I see, so you’re almost using it more like a guide instead of asking it to create something readymade.
LOVE: Exactly, and Martin thinks overall we should be encouraging the creators of AI tools to bake in this more-interactive design, just to ensure that our content continues to reflect as much of the quirkiness of humanity as possible.
PAVIN: That sounds all well and good. I can see how someone would improve their writing or coding by using this interactive method as opposed to just getting an output. But I’m thinking about what we were talking about earlier, which is that the main pitch for using these AI tools is to save time. Like, say you need to summarize a dense new policy that affects your team, but you don’t know when you’ll do that because you’re in back-to-back meetings. AI could be really useful in that way. You just feed it the text of the policy, a prompt, and you’re done. Wouldn’t doing this more-interactive method slow everything down and make it less appealing to people? Wouldn’t it defeat the purpose?
LOVE: You’re bringing up a great point, and it actually leads me to another thing I discussed with Martin. We’re a podcast focused on leadership, so I did want to make sure we addressed what executives should be thinking about when it comes to chatbots and other AI tools.
MARTIN: Understanding that AI is not just like this amazing machine that can do this amazing thing. AI is used by people, and the way introducing AI will help your company depends on how people choose to use the AI. If you put a lot of pressure, time pressure, on your employees, they will use the AI extremely differently than if you give them more time to be creative.
LOVE: It can be tempting to try to optimize productivity by having an AI do a task. But that approach might lead to consequences down the line that could be profoundly damaging to the quality of work.
PAVIN: So it really comes back to how people use these tools, which I feel like is a common theme in discussions about AI. We act like it’s just this magical tool, but at the end of the day, there are productive ways and harmful ways of using it.
LOVE: That’s Martin’s point. He definitely thinks that AI is something that leaders should encourage employees to use, but they shouldn’t lose sight of the fact that what they want is not whatever generic thing the AI spits out. They want something distinctive that only their employee could produce, just with the help of AI.
MARTIN: Yeah, that would be my main advice, to be very aware that it’s not the artificial intelligence—it’s the interaction between us and the artificial intelligence—that leads to the output.
PAVIN: Jess, before we wrap up here … I’m having flashbacks to the time we had ChatGPT write executive summaries for some of our articles, just to see what that would look like. And see if it would work for us since we have like a thousand articles in our archives: it would be great to get some algorithmic help! And it was not ... NOT ... work. It was like editing a writer who was really fast but needed a ton of hand-holding to get things sounding how we wanted them to sound. So I can see how it would be really important for managers to understand that—and to make sure their employees understand that, too. That they should see the AI as less of a magic wand and more of a digital coworker who can do a lot of grunt work but needs help taking things to the next level.
LOVE: That’s the idea.
PAVIN: Hm, well, Jess, that was fascinating. Thank you!
LOVE: Thanks!
[CREDITS]
PAVIN: This episode of The Insightful Leader was written by Andrew Meriwether. It was produced and edited by Laura Pavin, Jessica Love, Susie Allen, Fred Schmalz, Maja Kos and Blake Goble. It was mixed by Andrew Meriwether. Special thanks to Sebastien Martin. Want more Insightful Leader episodes? You can find us on iTunes, Spotify or our website: insight.kellogg.northwestern.edu. We’ll be back in a couple weeks with another episode of The Insightful Leader Podcast.