Finance & Accounting Sep 2, 2013
A Crystal Ball for the Courtroom
A new model predicts the outcome of securities fraud class action lawsuits
When shareholders contend that a firm has been fraudulently mismanaged and decide to sue en masse, a securities fraud class action is born.
“Class actions are big, big cases,” says Blake McShane, an assistant professor of marketing at the Kellogg School of Management. And as class actions go, those dealing with securities fraud are some of the biggest around: 40% of class actions pertain to securities fraud, and they involve 75% of all class action dollars.
Unsurprisingly, given their numbers and settlement sizes, securities fraud class actions have been studied extensively—from a legal angle, that is. “But as is typical in law, they’ve been looked at from a qualitative perspective,” McShane explains. Attorneys have conducted interviews and opined on cases but have not engaged in the quantitative analyses standard in many areas of business research. This has left all parties—plaintiffs, defendants, their attorneys, and especially the insurance companies on the hook for huge litigation payouts—wondering where they stand when a case is filed.
Building the Crystal Ball
So McShane, along with colleagues Oliver Watson of Juridgm, Inc., Tom Baker of the University of Pennsylvania Law School, and Sean Griffith of Fordham University School of Law, aimed to bring clarity to this uncertain state of affairs. Specifically, the researchers decided to build a statistical model that uses the information available the day a lawsuit is filed to predict both the probability of a settlement—which is to say, the probability that a given case will not be thrown out by a judge—as well as the amount of any potential settlement.
A model like this would allow interested parties to gauge their chances in court ahead of time. “Our goal was to do something prospective as opposed to retrospective,” says McShane. “Once the case has been decided, hey, just look in the newspaper.”
To build their model, the researchers examined around 1200 cases, of which two-thirds eventually settled. (For cases that settled, the median settlement amount was 16 million dollars, while the mean—more sensitive to outlier cases that drew huge windfalls—was 30 million dollars.) For each case, researchers took into account a wide range of information that would have been available as soon as the suit was filed: information about the plaintiffs, the firm, the firm’s stock market performance relative to the market as a whole and to its industry group, the nature of the alleged misconduct, and even the number of news stories that had been written about the firm in the previous year.
An interesting question, says McShane, is whether the two different stages of a case—settlement probability and settlement amount—are affected by different factors. “If you have some variables that you believe affect case outcomes … which stage of [the case] do they affect? Do they affect the ‘whether it settles’ stage, or the ‘how much it settles for given it settles’ stage? And maybe they affect both of them; maybe they affect one but not the other; maybe they affect one positively and one negatively.”
Important Predictors
So what did they find? A number of factors affect case outcomes. But perhaps surprisingly, there was very little overlap between the factors that affected one outcome measure versus the other.
“What we found was that cases with accounting violations alleged were more likely to settle.” — Blake McShane.
Sometimes this disparity seems reasonable. “There are some variables that we would a priori expect to affect one but not the other. One variable we have is market capitalization, which is how big is the company,” says McShane. Not shockingly, he continues, big companies tend to make bigger payouts when a case settles. But big companies are no more or less likely to settle than smaller companies. Fair enough.
Other times, though, the disparity is less easily explained. “What we found was that cases with accounting violations alleged were more likely to settle—which, okay, probably isn’t terribly surprising,” says McShane. “It’s one of these variables that says there’s something fishy going on, and where there’s smoke, there’s fire.” But strangely, these cases do not have larger settlement amounts. Once such a case gets past the judge, it seems, any alleged accounting violations do not seem to matter in in terms of how much the case settles for.
Spotting Black Swans
McShane suspects that the model could be a useful tool for anyone involved in a securities fraud class action suit. “The results are publically available—they’re in the paper. So you can read the paper and, based off the estimated model, you can gather data for your case and use the model to make a prediction,” says McShane.
Specifically, interested parties can get an indication of what kind of case they are looking at. Cases can be roughly lumped into one of four categories: 1) those that are highly likely to settle but only for a small amount of money, 2) those that are highly likely to settle for a large amount of money, 3) those that are unlikely to settle, and if they settle, only for a small sum, and 4) those that are unlikely to settle but hold the potential for a huge payout.
The first two scenarios are fairly predictable, and the third—though unpredictable—has very low stakes. It is the final scenario that can be the most dangerous. “If you observe cases like it again and again, you’re going to get real comfortable—you’re going to … think, ‘hey, this isn’t so bad.’ But eventually you’re going to be hit over the head with 50 million dollars and you’re going to say, ‘where did that come from?’” Being able to detect such cases—researchers call them “black swans”—on the docket allows everyone to plan accordingly.
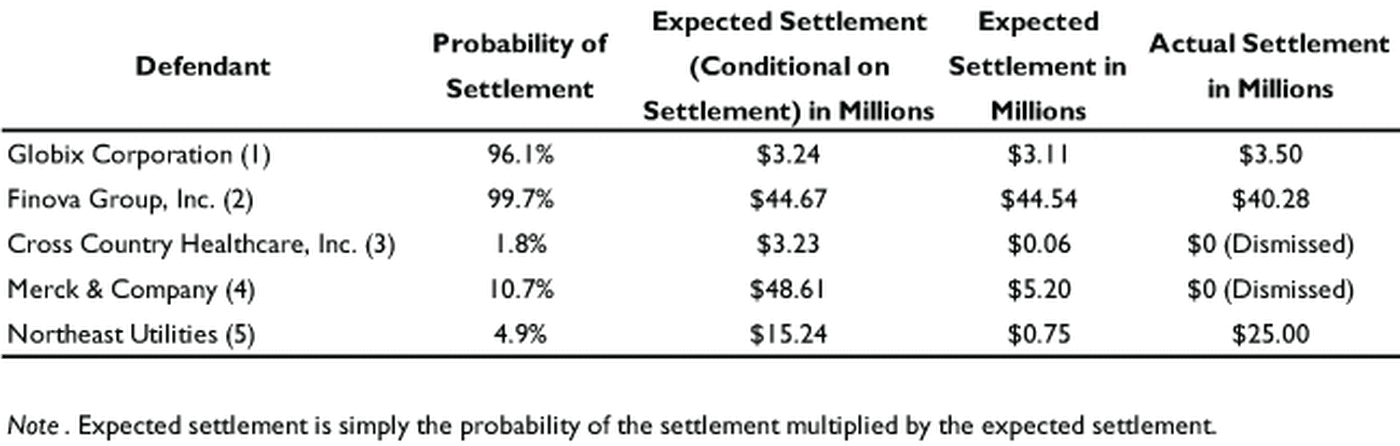
Other important findings? The researchers noted that a certain type of case—one hinging on a violation of the Securities and Exchange Commission Rule 10b-5—is less likely to settle than other cases. But when it settles, it tends to result in higher settlement amounts. “So you might as an attorney rationally be more willing to take these cases on even though you know there’s a lower likelihood of settling,” explains McShane. It might be worth it, just as when playing baseball “you might be willing to hack at the ball a little more and risk striking out for the chance that you’re going to hit a home run.”
Still, because the model is only probabilistic, it is far more useful for predicting case outcomes on aggregate than it is for any given case. This is why perhaps its most exciting application is its usefulness as a portfolio management tool for insurance companies. These companies know to expect a certain percentage of securities fraud litigation filings each year. Now, says McShane, they “can start thinking about, ‘How do I structure this portfolio? Are the risks associated with this case positively or negatively correlated with the risks associated with that case?’”
McShane, Blake, Oliver P. Watson, Tom Baker and Sean J. Griffith. 2012. “Predicting Securities Fraud Settlements and Amounts: A Hierarchical Bayesian Model of Federal Securities Class Action Lawsuits.” Journal of Empirical Legal Studies. 9(3): 482–510.