Strategy Nov 15, 2024
The Goldilocks Approach to Searching for Something New
Whether it’s the right dosage to a new drug or the right style of tennis racket for a novice player, it’s important to get your strategy right.
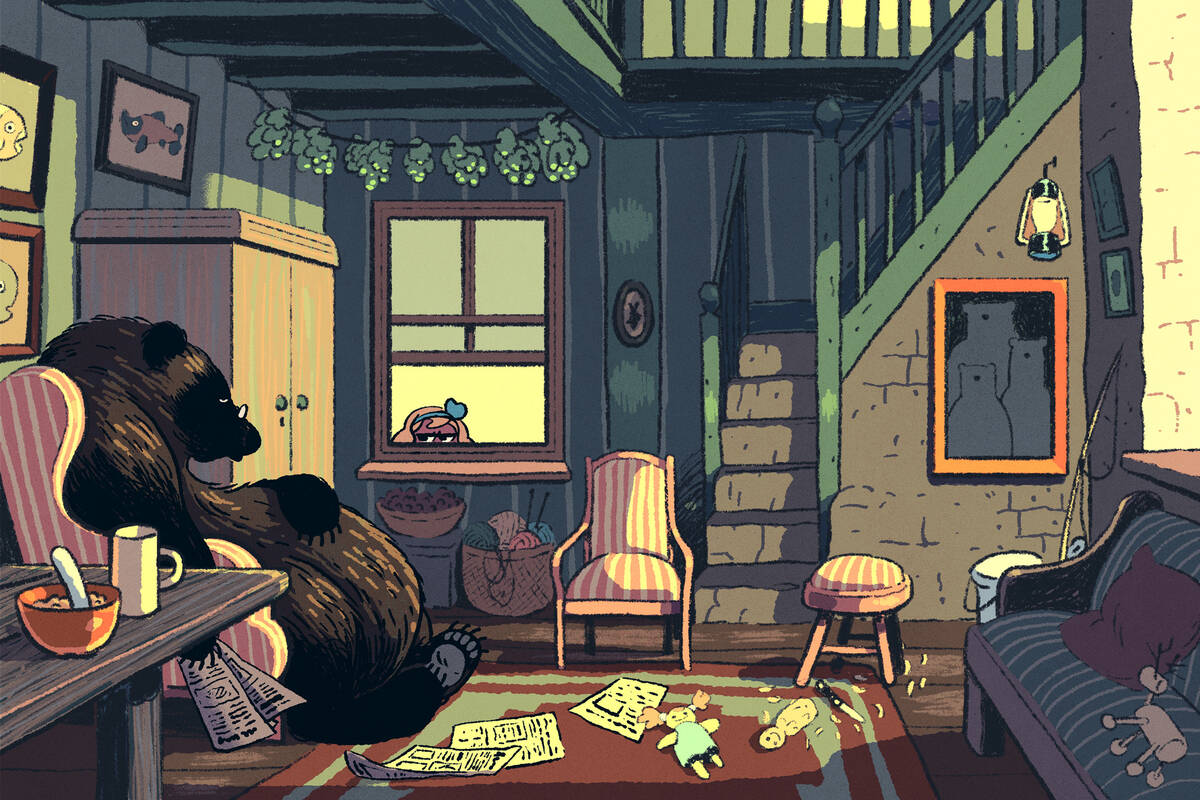
Michael Meier
“I’ll know it when I see it.”
It’s a common approach to use when we’re looking for something we want but don’t know how to perfectly describe. There’s just one problem: it only works when we’re in familiar territory. When searching for something genuinely novel—like a novice tennis player shopping for her first racket, or a pharmaceutical company determining the ideal dosage of a new drug—it’s hard to even recognize a good option in the first place. In these situations, what’s the best way to search, and how do we know when to stop?
“A lot of situations in everyday life look like these search problems,” says Suraj Malladi, an assistant professor of managerial economics and decision sciences at the Kellogg School. “What they have in common is that, in order to produce a good outcome, you have to try things—and that’s going to determine whether you keep searching or not, and what kind of thing you look for next.”
For instance, should the tennis player search for rackets that are very similar or very different from the first one she tries? Should the pharma lab experiment with similar dosage levels for its new drug, or try much higher or lower ones? And when is it ideal for either of them to call it quits?
Understanding both how people search and how they should search is a tall order. An important first step is to figure out the best possible search strategy. Malladi derived such a strategy in a mathematical model that describes how to use the information learned from each tryout to efficiently home in on a “sweet spot” of reasonably good options. As a research tool, this model could shed light on how people make decisions with imperfect information. It could also be converted into an algorithm to help optimize those decision processes in the real world.
“Search is costly—we can’t do it forever, so the goal isn’t to find the best thing that we possibly can,” Malladi explains. Instead, “the idea is that there are more and less painful ways” to learn where the good options tend to reside—“and I’m trying to solve for the least painful way.”
Avoiding pain, finding gain
Malladi’s model starts by assuming that a searcher—say, the pharmaceutical company seeking an ideal dosage for its new drug—wants to avoid two bad outcomes. The first is settling for a suboptimal dosage too soon, when a more effective one might be right around the corner. The second is going on a wild goose chase: wasting time and resources testing dosages that don’t work much better than the best one they’ve already tried.
“These are both bad strategies,” Malladi explains. “If I give up too soon, then I miss out on something really good. But if I try to learn the perfect dosage, it could take many years and billions of dollars.”
To capture how people learn from past discoveries and trade-off risks when searching unknown territory, the model makes two more assumptions about searching.
“Search is costly—we can’t do it forever, so the goal isn’t to find the best thing that we possibly can.”
—
Suraj Malladi
The first one is that, all things being equal, similar options will probably deliver similar outcomes—meaning that it isn’t always necessary to perform an exhaustive search to guess the payoffs. In the pharma lab’s case, this means that similar dosages of a drug will probably have similar effects. If they try a dose that works well, it stands to reason that they are in (or at least near) a sweet spot, and so similar choices may also work well or slightly better. But if the dosage works poorly, it wouldn’t make sense to try a similar dose in the same ballpark; searching elsewhere would likely produce better results.
The second assumption is that when searching the unknown, the objective is to find strategies that work reasonably well no matter what the searcher is up against. For example, imagine the pharma lab conducts its first test of a new drug, and a high dosage results in toxic side effects. What happens if they don’t try again? In a worst-case scenario, they gave up too soon and missed out on finding a low or intermediate dosage that would have yielded good results. On the other hand, if they planned to try five additional dosage levels, the worst-case scenario is that all these additional attempts still yield disappointing results. Which one of the myriad strategies the searcher should follow depends on whether the cost of missing out or the cost of additional search is higher.
The key, however, is that the model repeatedly updates what the worst-case scenario looks like based on the information gathered from each search and adjusts accordingly. Let’s say the pharmaceutical company has conducted many trials with a range of lower dosages, all of which resulted in similarly good—but not great—outcomes. If they stop searching now, a slightly better dosage might remain undiscovered. But since a neighborhood of good dosages has already been discovered, this worst-case scenario is still better than the cost of running additional trials—so it makes sense to stop searching.
“You’re repeatedly asking yourself how things can go wrong, and then hedging against those outcomes,” Malladi says. “In reality, you don’t actually expect the worst-case scenario to happen. But when you follow a procedure like this, even in the worst case, you’re doing pretty good—which means that in all other cases, you’re doing good as well.”
What smart searches look like
Malladi identified the best strategy and noticed it exhibited certain patterns. As the difficulty of finding a neighborhood of good options increases, the more sense it makes to keep searching—but only up to a certain point. Due to the high effort required to locate good outcomes, Malladi’s model implies that sometimes the optimal decision may be not to search at all.
“You might have a sense of how difficult the problem is, and that’s going to affect how you actually conduct the search,” Malladi explains. “If a drug company knows that a particular compound very quickly goes from ineffective to toxic with small changes in the dosage, finding a good dose is going to be like finding a needle in a haystack. You would have to do so much search to figure out where it is that you just say, ‘Nope, I’m not even trying.’”
For searches that are worth embarking on—that is, ones that have a sufficiently broad range of good options, if they exist at all—Malladi found that an optimal pattern always looks the same. As the searcher repeatedly tries options that hedge against a worst-case scenario, their choices naturally funnel them toward the sweet spot.
In the case of the pharma lab, for instance, imagine that the lab’s first attempt to find a good drug dosage returns bad news: the low dose they tried is ineffective. Since they’re obviously not near the sweet spot, the lab picks a much higher dosage to try next. Bad news again: this higher dosage turns out to be toxic. But these searches have now established a likely “floor” and “ceiling” that can help them avoid more bad outcomes. The lab tries a new dosage near the middle, and this time, the drug works better, indicating that they are closer to the sweet spot than before. “In these situations, the optimal search procedure is going to involve bouncing back and forth” between a narrowing range of options, Malladi explains.
This funneling pattern can be translated into an algorithm that could, in theory, be run on any computer. “If you’re actually interested in computing a solution to this search problem in various instances, you can do it,” he says.
Funneling toward success
Does this mean that Malladi has created a mechanical oracle that can tell tennis-racket shoppers and pharmaceutical companies how to find exactly what they’re looking for? Not quite. “But it does give us a framework for thinking about how people solve these problems,” he says. “And now there are things to check. Are people behaving in ways that are even close to this optimal approach?”
Malladi is currently running experiments to investigate that question. But in the meantime, his theoretical model could have practical applications. An online shopping platform, for example, could use it to offer more helpful suggestions to customers who are searching for products they don’t know much about.
“This is a good model to use when you’re discovering what your preferences are in a particular product category,” he explains. “If you’re trying to find the right digital camera, maybe earlier in your search session I’ll show you related products that are very different from what you’re looking at now. And later, when you’re homing in on what you want, the cameras I suggest can be more closely related.”
Firms hunting for innovation—whether it’s prototyping new products or developing new medications—could make use of this “funneling” model, too. “It says, ‘Here’s how you should actually sequence your experiments to zero in on a sweet spot,’” Malladi says. “You and I could come up with all sorts of approaches, but what’s the reason to pick one over the other? With this procedure, whatever happens in your search, you can’t go too far astray. And in that sense, it’s optimal.”
John Pavlus is a writer and filmmaker focusing on science, technology, and design topics. He lives in Portland, Oregon.
Malladi, Suraj. 2024. “Searching in the Dark and Learning Where to Look.” Working paper.