Economics Dec 3, 2024
Overnight Success? AI Has Been a Century in the Making.
For clues about the future of AI, it helps to understand the past.
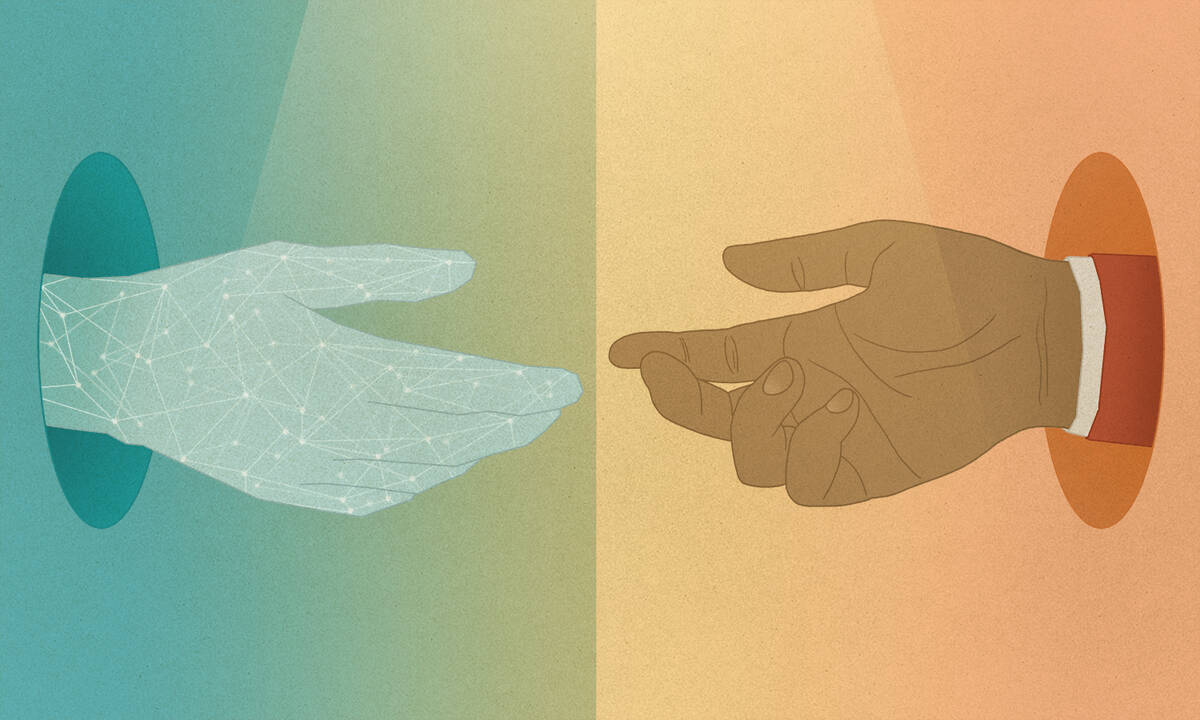
Lisa Röper
In 1956, a group of mathematicians and engineers gathered at Dartmouth College to devise an ambitious plan for building artificial intelligence. They wanted to program a computer to reason, plan, navigate, process natural language and translate it, perceive the world around it, and demonstrate creativity and intuition.
It seemed like a stretch at the time. But today, we have computers outplaying humans in chess, smartphones guiding us on trips, chatbots drafting essays, and apps translating languages almost instantaneously.
“We’ve accomplished a lot; we’ve come a long way in AI,” says Kellogg finance professor Sergio Rebelo. “But this progress only happened after many years of failures.”
In a recent webinar hosted by Kellogg Executive Education and Kellogg Insight, Rebelo traversed lessons from AI’s past through the lens of a macroeconomist to help us prepare for its rapidly expanding role in society—today and in the years to come.
1. Overnight successes can take decades to pull off
One of the early strategies in AI was to create expert systems. The goal was to feed a computer program as much knowledge as possible, making it an “expert” so it could use that information to perform relevant tasks.
The U.S. Defense Department employed this tactic during the Cold War, when it sought to create a machine that could quickly translate captured phrases from Russian into English. Computer scientists fed the machine a large number of words, rules, and definitions and then had it translate words one at a time.
All too often, however, the machine was unable to catch nuances in the languages and ended up producing inaccurate translations. For instance, the Biblical phrase, “the Spirit is willing, but the flesh is weak,” when translated into Russian and back into English, would turn into, “the whiskey is strong, but the meat is rotten.”
Decades later, the team at Google Translate was still using the same concept of an expert AI for its translations. The result, unsurprisingly, was that translations often ended up being too literal, missing the subtleties of language. It wasn’t until 2016, when Google abandoned this approach, that its AI translation took off. The team leveraged neural networks to process entire sentences at once, using context to refine its translations.
It wasn’t an overnight success, Rebelo says, but instead, years of trial and error have brought AI translation to where it is today. “And the success that we’re seeing now with generative AI is a bit of the same thing. It’s a seemingly overnight success that was more than 50 years in the making.”
Rebelo adds that much of this progress has been possible because of the government’s long-standing commitment to funding AI research.
“We are where we are because, despite failing for 50 years, the government has kept funding this research,” he says.
2. Intuition can guide you toward smart risks
Early in her career, when she was an assistant professor at Stanford, computer scientist Fei-Fei Li had an idea about what was holding AI back.
Her intuition told her that “what was missing was data,” Rebelo says. “And that if you had more data and the computational power to process it, you could unlock potentially magical results.”
Feeling inspired, she poured all her resources into pursuing this hunch. So she, along with her PhD students, began hand-labeling images to create a large-enough dataset on which to train algorithms, hoping that AI might eventually come to understand images.
“She decided to do something extraordinarily risky,” Rebelo says, dedicating about 2.5 years to the task rather than focusing on more surefire projects that might have otherwise helped her more easily secure tenure.
This gamble ultimately led to the creation of ImageNet, a public dataset containing millions of images. By relying on this dataset, a different team of computer scientists—led by the “godfather of AI,” Geoffrey Hinton—was able to develop an algorithm that could label images, describing their content. The team had their algorithm analyze a fresh set of images at an AI competition in 2012. It completely blew the other algorithms out of the water.
“The improvement was absolutely dramatic,” Rebelo says. “This was an amazing breakthrough, a watershed moment for modern AI.”
From that point on, the race was on to get enough data to feed these insatiable algorithms. People came to understand that advancing AI depended less on building knowledge into their algorithms and more on scaling them through big data.
And the advancement in AI that followed soon thereafter was made possible because one early career scientist decided to take a risk.
3. Did we say stay the course for decades? Try a century
Yet not all ventures into AI have borne fruit so quickly, Rebelo notes. Some have been confronted by significant hurdles and spanned decades, if not a century, to progress.
The mathematician Andrey Markov, who had spent years working on an early language model, wrote a letter to the Academy of Sciences of St. Petersburg in 1921 to tell them he had a breakthrough.
“There’s a lot of anxiety about people being replaced by AI. I’m going to tell you—the first people who will be replaced are those who don’t know how to use AI. And they will be replaced not by AI but by people who know how to use AI.”
—
Sergio Rebelo
He had been working on an algorithm designed to write poetry. But there was a problem. He didn’t have the means to physically get to the Academy to present his work. The Academy sent him a pair of boots to help, but they were the wrong size. Markov never made it to the presentation. About a year later, he died.
Nearly a century after that, in 2017, a team of Google computer scientists solved the problem tackled by Markov using a new form of neural network (the transformer) that eventually served as the foundation for today’s popular large language models (LLMs), like ChatGPT.
“Maybe the breakthroughs we’re having now [with LLMs] could have happened much sooner if we had not lost that paper in 1921,” Rebelo says. “Be that as it may … transformers have now gone on to produce amazing results.”
Despite all of the growth, AI still has plenty of issues that need to be solved. Hallucination, in which AI makes up part of the information it provides, is a common one. This flaw has intimidated many groups. Some law firms, for example, have gone so far as to forbid their employees from using LLMs for their work after a lawyer was caught handing a judge an AI-written brief full of made-up cases.
But from Rebelo’s viewpoint, deciding to stop using AI tools out of fear is a mistake—one that will only set people further back.
“There’s a lot of anxiety about people being replaced by AI,” Rebelo says. “I’m going to tell you—the first people who will be replaced are those who don’t know how to use AI. And they will be replaced not by AI but by people who know how to use AI.”
4. Be mindful of hype
Among the many milestones in AI development, “the most impressive achievements so far are in biology,” Rebelo says, referring to the application of AI in understanding protein structures.
Until 2019, scientists had determined the structure of about 170,000 proteins, which was a huge achievement given that folding a single protein was considered a years-long project worthy of a PhD thesis. However, in 2020, the AI program AlphaFold determined the unique structures of more than 200 million proteins.
“Clearly, we are at the dawn of something new,” Rebelo says. “At the same time, there’s a lot of hype and snake oil.”
There’s this impression that “AI is some kind of magic, one-size-fits-all tool,” he continues. “The reality is very different.”
Take ChatGPT, for example. To the regular user’s eyes, it looks like a singular, complex algorithm capable of so much—from all manner of text generation to audio processing. But behind the scenes, it’s a collection of specialized algorithms that are individually great at performing a specific task but terrible at most others.
“Sometimes people’s perception is that AI looks like a series of beautiful, shiny copper pipes,” Rebelo says, “when, in fact, it is more like my basement, where everything is fixed with duct tape. There’s a lot of duct-taping in AI.”
There are also worries that AI is hitting a wall and that OpenAI’s new LLM, Orion, isn’t necessarily better than its predecessor, ChatGPT. Similar rumors exist about Google’s Gemini and Anthropic’s latest version of its chatbot, Claude.
“Whether data scaling will continue to be a source of great improvement in AI, or we are entering an age of diminishing returns … no one knows,” Rebelo says.
Still, that’s no reason not to celebrate what we have achieved so far, he says, because “what is true is that the recent achievement has been quite amazing.”
Abraham Kim is senior research editor of Kellogg Insight.