Marketing Sep 5, 2019
Is Your Digital-Advertising Strategy Paying Off?
Brands are demanding evidence that campaigns are working. Here’s what they should be asking.
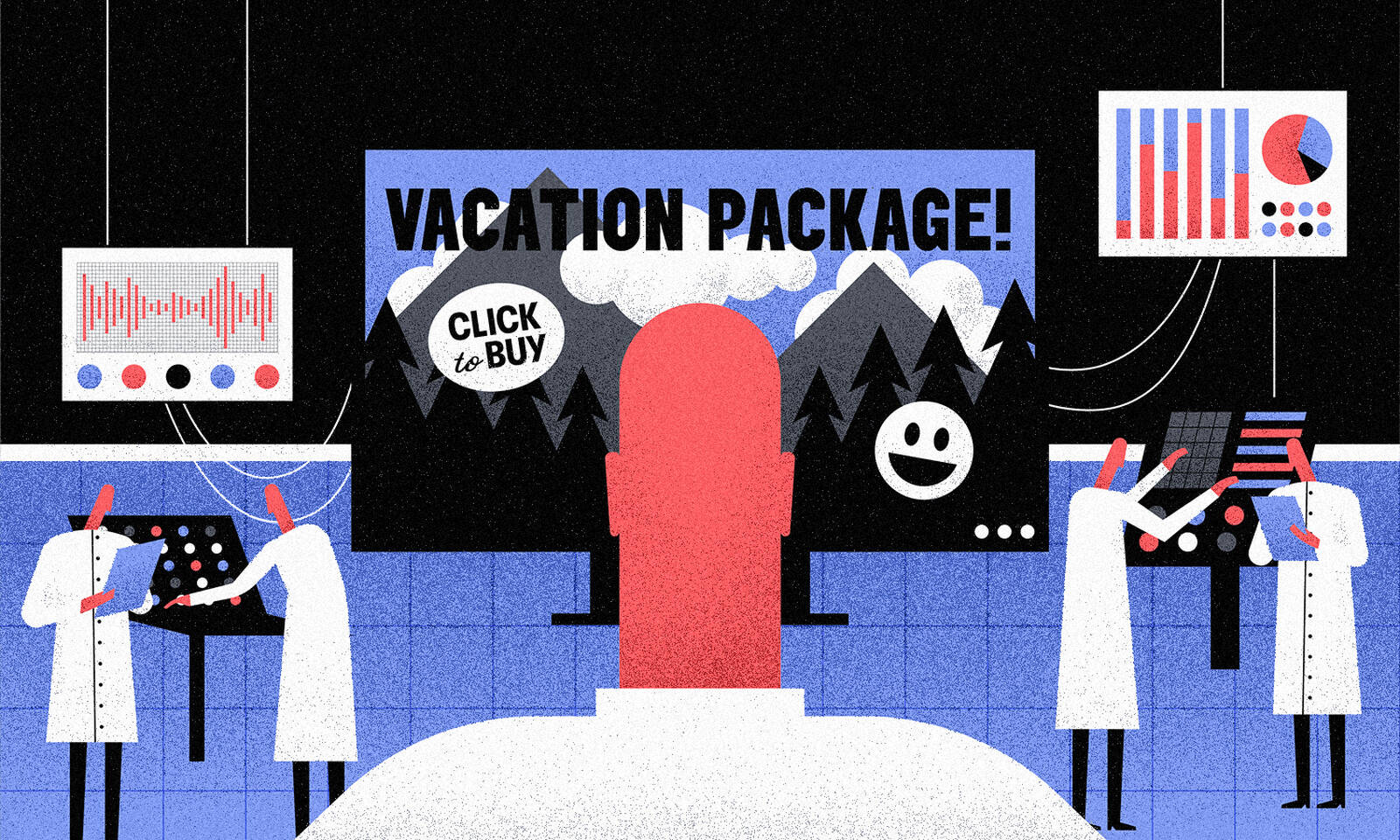
Riley Mann
Digital advertising continues its march toward dominance. In 2017, digital spending surpassed television advertising in the U.S. for the first time; by 2019, it overtook advertising on all traditional channels. And some estimates suggest that by 2023, digital could account for as much as two-thirds of ad spending.
This shift has, of course, impacted the industries and channels that have long relied on advertisers’ dollars. But it has also drastically reshaped marketing itself, giving marketers an unparalleled amount of data upon which to make decisions—as well as a new mandate to deliver quantifiable results.
The days when a firm might simply entrust an agency with a fixed advertising budget and hope for the best are long gone, says Brett Gordon, an associate professor of marketing. “With the digital revolution, better measurement has become more feasible, and that has created more of an onus on the advertising ecosystem to demonstrate value.”
So how can companies make sure they are getting the most from their digital spending? We spoke with Gordon about the importance of running randomized experiments, the analytics tools that are available to help advertisers today, and the trends that are most likely to change digital advertising in the future.
This interview was edited for length and clarity.
Learn more from Brett Gordon in Kellogg Executive Education’s Strategic Marketing Communications program.
Insight: As digital advertising is maturing, advertisers are increasingly demanding to know whether they are getting a return on their investment. What questions should CMOs be asking themselves to ensure they are making smart decisions?
Gordon: I think the number one thing they should always be asking is: Are they getting what they want for their money? Is it worth it? And how do they know it is worth it?
This is where the importance of correct measurement comes in. As an advertiser, you are the one who cares the most about whether the measurement is being done correctly or not. Someone selling you ads may have incentives that don’t align with yours.
In many ways, measurement is very, very simple. To measure anything, you need a control group, where you are either not running the campaign or running it at a much lower volume. And you need that control group to be as similar as possible to the group where you are actually running the campaign. So if someone is telling you about the effectiveness of your campaign, you should be able to ask: What is the basis of comparison? What was the control and why should I believe that the control is similar to the people who were in the test? The more similar you can believe those are, the more valid the results are.
Insight: That makes sense. But can you explain just how similar a control group needs to be in order to reliably measure whether your campaign is working? On the one hand, there are randomized controlled trials—experiments with true control groups, not unlike ones you would find in academic or medical research. But there also exist a plethora of alternative approaches, workarounds really, such as creating a control group (or market) that is similar in a variety of ways to the one that sees the ad, or comparing the same group before versus after a campaign.
Brands are looking closely at their marketing budgets and asking, “For every dollar I spend, am I getting more than a dollar back? And if that’s not the case, then should I be spending it elsewhere?”
— Brett Gordon
Gordon: We have research we did with Facebook that looks at this. We were wondering if companies could rely on statistical models using observational data, i.e., not data generated from an experiment but simply data collected in the normal course of running a campaign. Our question was: How well do these observational methods do? And the beauty of partnering with Facebook was they let us directly compare randomized experiments to several observational approaches to assess whether they were a good substitute. And by and large, we found that unfortunately you couldn’t rely on the observational techniques to recover anything close to the true experimental effect of the ad campaigns.
Facebook should have been a best-case scenario for these other approaches, because we had a very controlled environment and lots of information about individual users, their exposure to ads, and the purchases or conversions on or around the platform. So if we couldn’t recover the actual effect using observational techniques in our nice, clean, walled garden, it would be much harder, we think, for someone else to use these observational techniques outside of this platform.
Insight: How difficult is it to run these randomized controlled trials on these platforms? Is it always worth the time or money?
Gordon: These are much easier to run than they used to be. Different platforms have started to make a number of experimentation features freely available to advertisers, such as “ghost ads” testing on Google or Conversion Lift on Facebook. Companies have done a lot, I think, to make them as user friendly as possible.
But it really comes down to a different question, which is: What do you think you know versus what do you think you don’t know?
As an advertiser, if you are absolutely positive that this campaign you are about to run is going to make you a lot of money, then you may not want to run an experiment. An experiment entails not showing that campaign to some people. If you are sure it is going to make you money, you want to show it to everyone possible: you don’t want that control group to not see your campaign.
On the other hand, if you’re uncertain, or you want to know how effective the campaign will be, then you need to have a control group. Only by having a control group do you actually know the counterfactual: what would have happened had you not run the campaign.
Insight: OK, so you run an experiment with a randomized control group, and now you know how effective that particular campaign is. What do you do next? You don’t have that same level of data about the other channels you may be interested in advertising in, such as print or television.
Gordon: That’s right. A bigger challenge is definitely knowing what to do with the information you get. I think that’s where a lot of advertisers haven’t cracked the nut yet.
Brands are looking closely at their marketing budgets and asking, “For every dollar I spend, am I getting more than a dollar back? And if that’s not the case, then should I be spending it elsewhere?” But now they’re spending it in lots of different buckets, and in some of those buckets it’s easier to measure the returns than in others. So how do you, as a brand, incorporate this ambiguity into your decision-making?
This is where your own intuition and instinct come in. But by virtue of what you know about how your customers behave and how the channel behaves and who is going to see the campaign or not—data you should be able to get—you can have a sense of what might work. You will also be able to look at outcomes after the fact. Do you see a bump in your business from the right channel?
Still, now you have to weigh, say, a guaranteed 20 percent return from a digital campaign against something that you think was positive but could be more or could be less. That’s why, in the end, people diversify their allocations, because they know they need to take a portfolio approach. Marketing spending is essentially a portfolio-optimization problem, except it’s in some ways harder than finance because we can’t always measure the return even after the fact.
Insight: How will advances in machine learning change digital advertising?
Gordon: Machine learning has already changed advertising by improving predictions about how someone will respond to an ad. Machine learning is very, very good at problems where you have lots of information and are trying to make a prediction, such as will someone click on an ad if I show it to them. And machine learning is very good about doing this quickly and on a large scale. So it is already part of the plumbing now in a lot of digital-advertising campaigns, whether people know it or not.
Insight: Are there other trends marketing leaders should keep an eye on?
Gordon: Absolutely. First is the emergence of other companies in the advertising space. Take Amazon. There’s long been this duopoly of Google and Facebook, with 37 percent and 22 percent of the market, respectively. Amazon is still a long way away in terms of market share—at about 8 percent--but they have a lot of momentum. Being a retailer, they are the last touchpoint. And so as an advertiser, there is great value in advertising on their platform, because you are so close to the buying stage that the targeting capabilities become quite compelling. Or imagine if Netflix all of a sudden got into the ad business. They have fantastic customer data, and they’re technologically very capable as a company. I think a lot of advertisers would love to be able to advertise on Netflix.
Second is GDPR. So far, the broader implications of this regulation have not really been felt, because implementation has been so slow and varied across websites. Many websites don’t follow the letter of the law or the intent of the law. I think the near-term effects—which haven’t been much—are not indicative of what the long-run effects are going to be.
Third, I think another unknown in terms of privacy is what Apple might do. Apple has lots of control over the information passed to advertisers on iPhones or in Apple apps. Companies that collect cookie data normally see all of people’s movements across the web, which allows for increased targeting and optimization. But Apple may want to break up the link between people seeing an ad and people buying something. This is already part of an experimental feature in Safari, and they are in the early stages of proposing it through the W3C, which develops standards for the web.
Apple can do this because they don’t currently rely on ads for a big part of their business. So in some ways, anything that they do to make it harder for the rest of the ad ecosystem to operate is probably a win for them.