Data Analytics Marketing Dec 2, 2019
How Companies Can Mine Online Reviews for Product-Development Gold
The right techniques can uncover valuable insights in user-generated content.
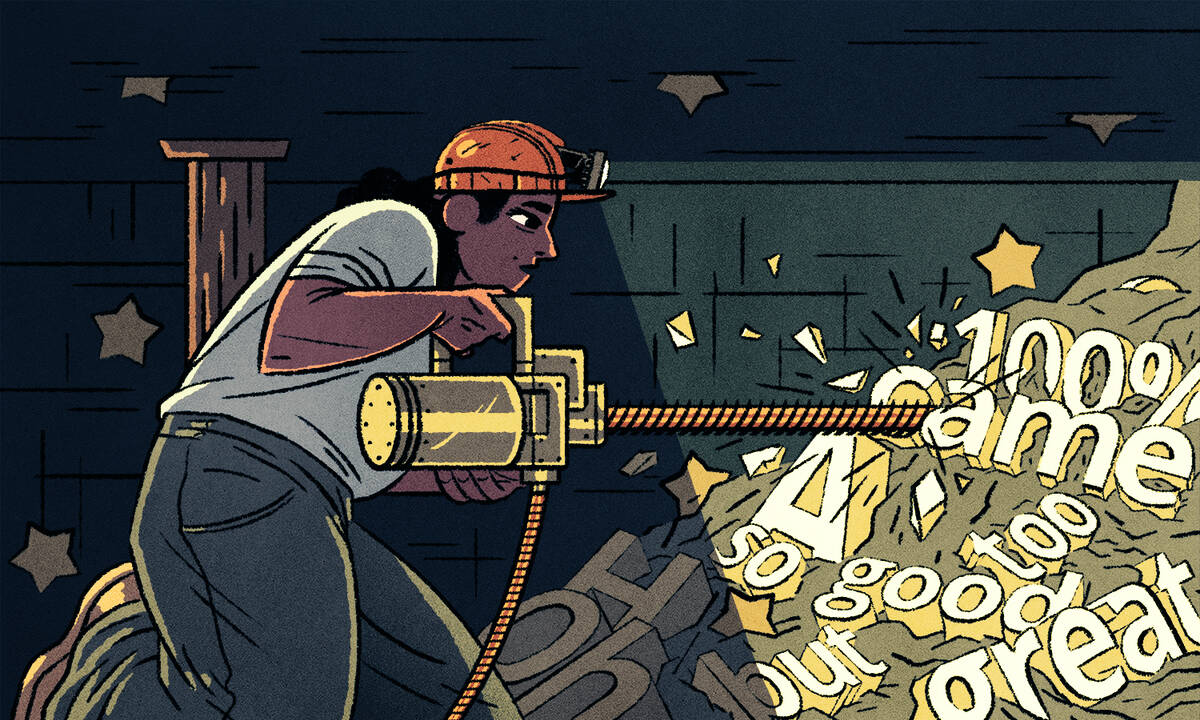
Michael Meier
Consumer products rarely remain static. They continually evolve based on customer needs.
Take the electric razor. About a century ago, one of the first models—the Vibro-Shave—entered the U.S. market. This razor, which was marketed to both men and women, had a vibrating handle that moved a blade from side to side. And, if you were so inclined, you could swap out the top for a massage head, to “coax” away your wrinkles.
Needless to say, razors have changed over the years.
So how does product development ordinarily occur? Companies have long relied on market research to determine how customers are using their products and whether they have underlying needs that a new feature or innovation might meet. Much of this research has traditionally involved interviews or focus groups with customers, who share how they use a product, what they like, and what they don’t. Companies then synthesize this feedback, to determine if customer needs are being met, and act on this knowledge.
But interviews and focus groups are expensive, and they can take an enormous amount of time, says Artem Timoshenko, an assistant professor of marketing at Kellogg. “Being on the market with a new razor half a year before your competitor gives you the edge.”
So Artem Timoshenko and his colleague John Hauser of MIT Sloan wondered whether it was possible to glean similar insights about customer needs from existing customer feedback—namely, user-generated content like Amazon reviews or social-media data.
They had two specific questions: First, could professional market-research analysts extract useful information from these reviews? And second, could machine-learning algorithms enable them to do so more efficiently?
Mining Product Reviews
To address this first question, the researchers brought in a marketing-consulting company called Applied Marketing Science, Inc. (AMS). AMS has over twenty years of experience in market research and customer-need elicitation, and they had recently conducted a for-client interview-based study of customer needs for oral-care products.
“It was very convenient from both a business and a research perspective,” Timoshenko explains, as toothbrushes represent a fairly standard product category and one with plentiful Amazon reviews. Moreover, AMS was excited about the researchers’ questions, and the company was eager to partner.
When it comes to oral-care products, many customers report needs that are fairly straightforward: the products need to keep their teeth clean and white, keep their gums healthy, and not damage any previous dental work. But other customers might mention less expected needs, such as knowing how long to spend on various parts of their mouth during their oral-care routine. This might lead to product ideas, such as toothbrushes that beep at timed intervals or shut down after a certain number of minutes.
The experiential interviews conducted by AMS revealed 86 different customer needs for oral-care products, a typical number for such a product category. The goal of analyzing these customer needs is to find a hidden gem: a need that is very important, but that existing products do not meet well.
“At least for some categories, we are able to fully eliminate the need to conduct interviews and focus groups.”
— Artem Timoshenko
To determine whether marketers can glean the same kind of information about customer needs—and potential hidden gems—from user-generated online reviews as they can from interviews and focus groups, the researchers randomly selected a subset of Amazon reviews for oral-care products and provided that to a group of analysts at AMS. These analysts were not the ones who had collected or analyzed the customer interviews, but they were similarly trained. Each of the reviews in the subset was presented to the analysts in its entirety, and together the reviews added up to 12,000 sentences—which took the analysts approximately the same time to review as a standard set of 20–25 experiential-interview transcripts.
Going into the study, Timoshenko and Hauser thought that the Amazon reviews might have some advantages over traditional customer interviews. For example, perhaps they offered access to a population of customers who were unlikely to participate in a focus group.
“We could imagine that, if a company’s located in Boston, they would mostly interview Bostonians,” says Timoshenko. “But maybe people in other areas have different product experiences and usage models.”
Another possible advantage is that customers tend to write online reviews immediately after using something. Participants in a focus group, on the other hand, might have used the product a month or two before they are interviewed and have already forgotten key parts of their experience.
However, the researchers also suspected that online reviews might have a major disadvantage. Specifically, “there is a lot of research suggesting that online reviews are skewed toward extremely positive or extremely negative,” says Timoshenko. “So we might be missing some of the customer needs that are usually expressed in more neutral language.”
For instance, the fact that a toothbrush actually cleans teeth—an important but by no means thrilling use—might not be the kind of thing that a customer would bother mentioning. That was a major concern, as articulating the entire set of customer needs can help product-management teams to identify new product opportunities, even when some of the customer needs are not surprising ex post.
So what did the researchers find? First, almost all—97 percent—of the customer needs identified in the interviews and focus groups were also found in the Amazon reviews.
“That immediately suggests that, at least for some categories, we are able to fully eliminate the need to conduct interviews and focus groups,” says Timoshenko. “And that is the most time-consuming part of market research for customer needs.”
The second finding was that the Amazon reviews contained eight additional customer needs (nearly 10 percent of the total) that were not mentioned during the interviews. These were not materially different from those that were mentioned by customers—they appeared to be just as important to customers and useful for future product development—suggesting that analyzing user-generated reviews could provide a more exhaustive insight into customer needs.
Timoshenko suspects that, if additional interviews and focus groups had been conducted, these needs would have eventually emerged. “But doubling the number of interviews you conduct is much more expensive, in money and time, than just doubling the amount of online content we review.”
Machines Aiding Humans
Next, the researchers attempted to see whether they could use machine learning to make the human analysts more efficient. Specifically, they built an algorithm to “prescreen” the reviews, weeding out less helpful ones so that analysts could make more productive use of their time.
The researchers trained an algorithm to prescreen the reviews in two ways: it removed non-informative sentences, and it reduced redundant ones. Non-informative sentences, which make up nearly half of all of the sentences in the corpus, might simply say, “My son loves this product”—a perfectly legitimate sentiment, but not one that will lead to product innovation. Redundant reviews, also prevalent in the corpus, mention the same deficit or perk over and over again.
The researchers found that the prescreening by their algorithm allowed the analysts to find the same number of customer needs in about 20 percent fewer sentences.
“This was the proof of concept,” says Timoshenko. He is confident that with more experience and engineering, efficiencies would continue to increase, just as the methods for traditional-interview-based market research have improved over years of practice.
To that end, the researchers have made their code freely available to companies and are eager to learn about how it is being further developed and applied by companies in different industries.
One company in the food industry, for instance, has used the researchers’ methods and found that they identify very different kinds of customer needs depending on whether they search online reviews or social-media data.
Timoshenko says this highlights the fact that, as multiple sources of feedback are considered, the need for machine-learning tools will only grow.
“There is even more need for preprocessing this information,” he says. “Because there are millions of Amazon reviews for a particular product—but if you want to combine that with the social-media data and online reviews from other sources, it just blows up the amount of content you have to process. And that makes machine learning very important.”
Unexpected Benefits
In doing their research, Timoshenko and Hauser found that analyzing user-generated content has another, quite unexpected, advantage over traditional interviews and focus groups: the ability to “follow up” on an intriguing customer comment or need in order to dig deeper.
In a traditional-interview setting, he explains, “you don’t have the chance to call back the same interviewee and talk about this experience. It’s a lost opportunity.”
With user-generated content, on the other hand, you actually can explore further. With an interesting lead in mind, you might go back to the entire corpus of thousands of reviews to search for additional clues. “You don’t go to exactly the same customer review, but you could look for the keyword, or a particular phrase, or the particular experience,” Timoshenko says.
Overall, he wants marketers to understand that machine learning can be a powerful tool—not just for replacing human intelligence, but for augmenting it.
“One of the big breakthroughs in this research was when we agreed on the idea that machine learning cannot solve all the challenges of this process,” says Timoshenko. “Most people, when they think about machine learning, they look for completely automated solutions. It appears that humans are just much better, naturally, in some tasks than machines. And they will stay better in the foreseeable future. And formulating customer needs is one of these tasks.”
A customer might say, “I don’t like this toothbrush because it doesn’t have a 30-second timer.” But the underlying customer need is wanting to know how much time to spend on various parts of your dental routine.
“It’s very abstract. It’s very conceptual what the customer really wants. So this step is better done by humans, who can really learn and understand the human experience of other customers.”