Operations Mar 10, 2017
How to Predict Demand for Your New Product
Relying on manager expertise and market research may not be enough.
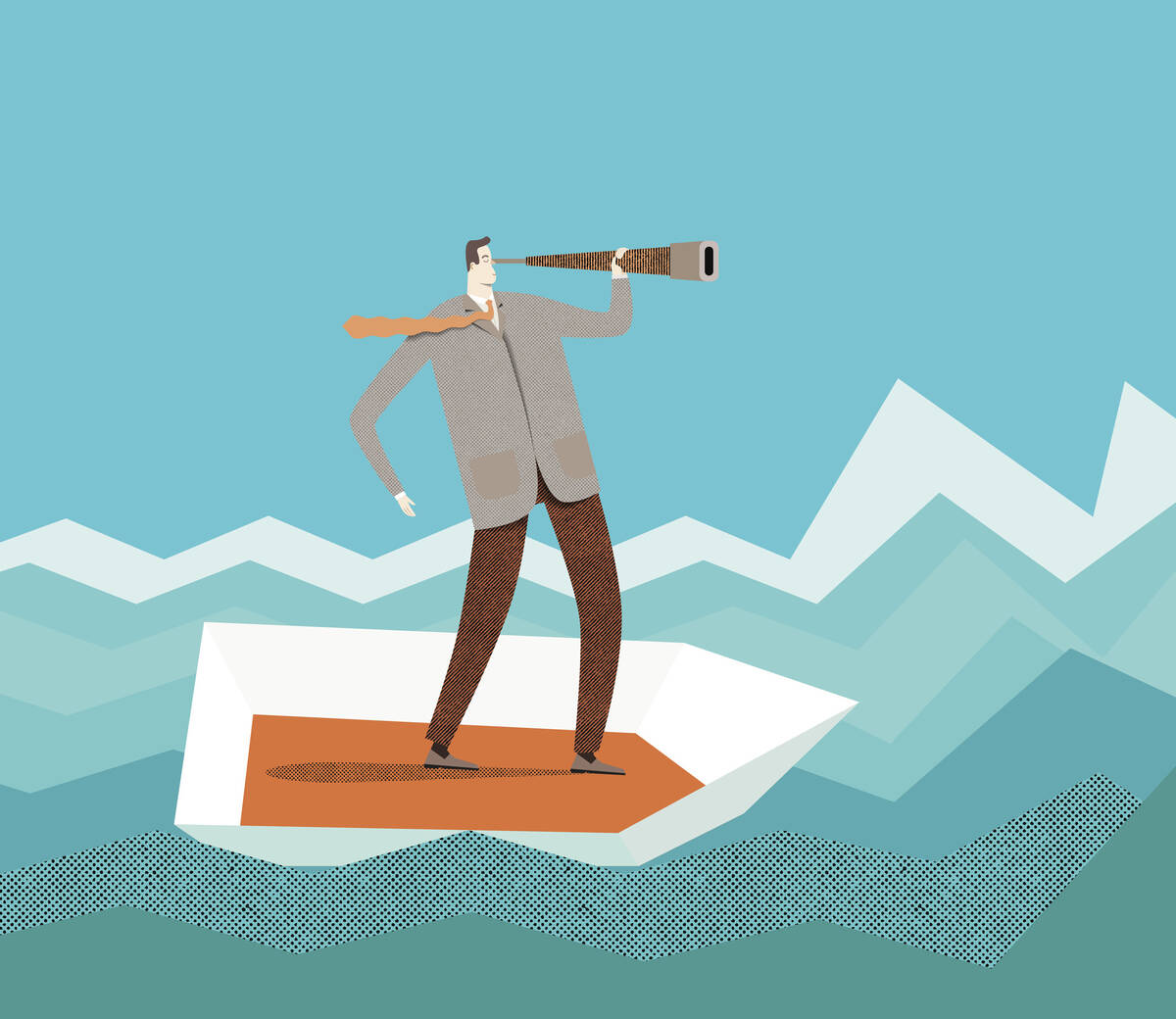
grivina via iStock
Launching the next line of laptops, routers, or surveillance cameras is critical for a tech company that does not want to be left behind.
But that doesn’t make it any easier to turn a profit off of them.
One of the biggest challenges that companies face is predicting demand for new products over time. Overestimate it, and risk warehouses full of excess inventory. Underestimate it, and your customers could leave empty handed—or you might be left with a hefty bill for expedited delivery.
“Imagine you have a crystal ball and you know exactly how demand for your product will go up or down, month by month,” says Jan Van Mieghem,
a professor of operations at the Kellogg School. “That would make it
very easy to prepare to meet demand, because if you know your lead
times, you just use your crystal ball to source the right number of
units from the cheapest source on time, and you can satisfy 100% of
demand with no waste. That is the Holy Grail.”
But crystal balls are difficult to come by. So Van Mieghem decided to find the next best thing.
In a new study led by Van Mieghem and Doug Thomas of Penn State University, researchers partnered with Dell to analyze sales data from over a hundred of the company’s products. While companies often rely primarily on managers’ experience and market research, the team found that companies like Dell could use historical data from previous products to improve forecasting accuracy on new products by as much as 9%. This translates into millions of dollars of savings.
The Cost of Getting Life-Cycle Forecasts Wrong
New products represent 27% of sales across all industries. Given the stakes, it should come as no surprise that businesses invest in forecasting the lifecycles of their products.
“Small forecasting errors can mean a big hit on profits,” says Van Mieghem. “When companies like Dell face stock-outs, they do emergency air shipments or use a faster local source such as Mexico instead of China. Those options are more expensive than their traditional sourcing.”
For existing products, companies generally use data on previous sales to create, or at least influence, a forecast for the next sales period.
But new products are trickier, and previous research has found that most firms do not rely primarily on historic sales data. Instead, they lean heavily on qualitative market research, or the opinions of executives. “In that situation,” Van Mieghem says, “you typically rely on management insight and experience, which is not really data-driven.”
Yes, there is the occasional product that is truly groundbreaking. But what if most new computers or cameras were not truly new? What if, despite an upgrade here, or a new feature there, they resembled past products in key ways? Wouldn’t this suggest that historical data for other products could be used in new product forecasting?
Van Mieghem and Thomas teamed up with Kejia Hu of Kellogg, Jason Acimovic of Penn State, and Francisco Erize of Dell to find out. The researchers came up with the idea of creating clusters of products that had similar product life-cycle (PLC) curves—literally the shape of the curve when the product’s demand over time is made into a graph. Then they could make predictions about which “cluster” a new product would fall in, based on the previous products it most resembled.
“The idea is that for each product cluster we can find the product life-cycle curve that fits it best and use this curve to forecast demand for the new product,” Van Mieghem says. “If a new product is exactly like an old one, you can use the curve just for that product to predict sales. But if it doesn’t have an exact match, you can use the curve for the cluster it fits into.”
What if most new computers or cameras were not truly new? What if, despite an upgrade here, or a new feature there, they resembled past products in key ways?
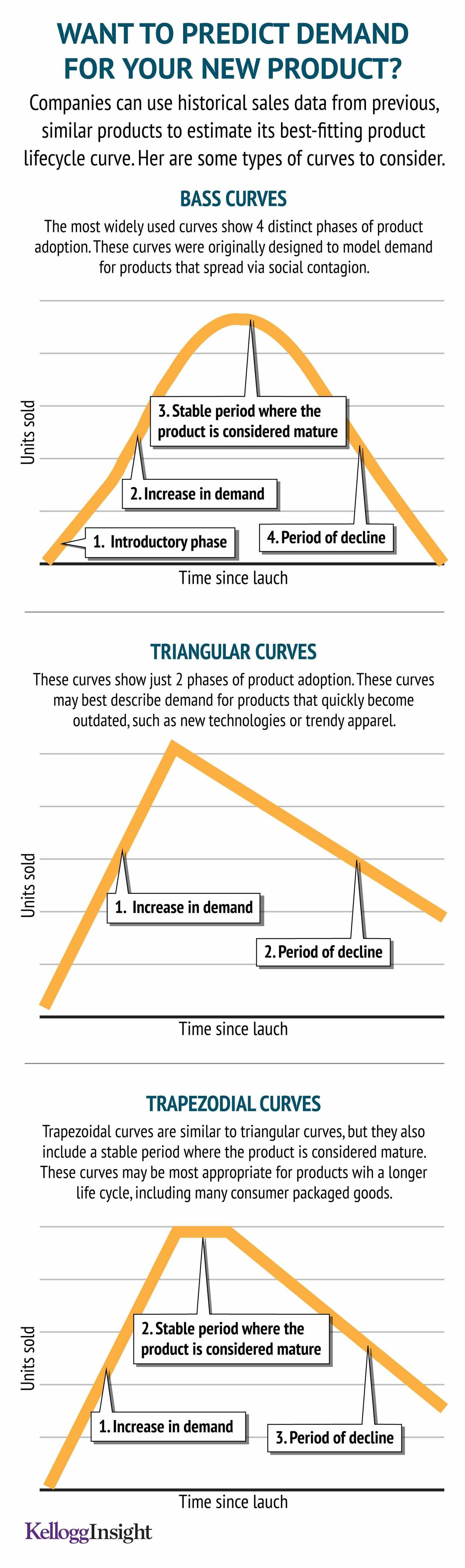
Comparing Curves
Armed with data on 133 Dell computer products, the researchers first worked to find out which product life-cycle curves best fit historical sales patterns, starting with the historical sales of individual products.
Some of the curves they considered were more typical, having four phases: an introduction, a ramp-up of demand, a more stable period in which the product is considered “mature,” and finally decline. The researchers also included curves that were more linear in their analysis. These curves resembled triangles and trapezoids. Their goal was to find a curve that fit the historic data well—but not so well that the curve was too specific to that particular product.
The researchers found that the best product life-cycle curve for the majority of the products was not curved at all. It was a triangle. “Demand goes up, then it goes down,” Van Mieghem says.
This suggests that these products experience very little in the way of a mature period. Which makes sense for electronics, since there is generally a newer, faster, flashier model out before the previous product has run its course.
“This is very attractive managerially,” he says. “If you’re a manager trying to predict demand for a new product and I tell you it’s triangular in shape, you only need to estimate three numbers: how long you think the product will sell for, when the peak sales will occur, and how high the peak will be.”
Jan Van Mieghem teaches programs in operations strategy, lean operations, and supply chain management through Kellogg Executive Education.
Next the researchers created clusters of products with similar life cycle curves and found the optimal life-cycle curve for the entire cluster. They then used this curve—in addition to other information about the product that a company might have, such as the season of launch, the planned end of life, and the estimate of total PLC demand—to scale the curve and recreate a demand forecast for each product.
A Better Way
Overall, the researchers’ forecasts were about 9% more accurate (in terms of Mean Absolute Error, or MAE) when compared against the historical data than Dell’s original forecasts. This is a sizable improvement that could drive transportation and inventory savings of $2–$6 per unit on millions of products a year.
Van Mieghem believes the triangular life-cycle curve should be a good fit for any product without a significant maturity phase, such as clothes or accessories pegged to the latest fashions, as well as electronics. In addition, the “cluster” approach that the researchers used to identify the best curve should be effective for a wide range of new products that resemble older ones.
The key, according to Van Mieghem, is that companies like Dell can achieve greater forecast accuracy by combining data-driven approaches with knowledge and business insight from demand planners.
“Using historical data of predecessors, our methodology will suggest the most appropriate shape of the life cycle curve for a new product in a particular business. A planner can then use that data-driven product lifecycle curve as a starting point and adjust with their particular insights about the specific product, such as when they know about an upcoming promotional push.”