Operations Innovation Apr 1, 2025
The Hidden Cost of Successful Experiments
As companies innovate, the resulting complexity makes further growth more challenging.
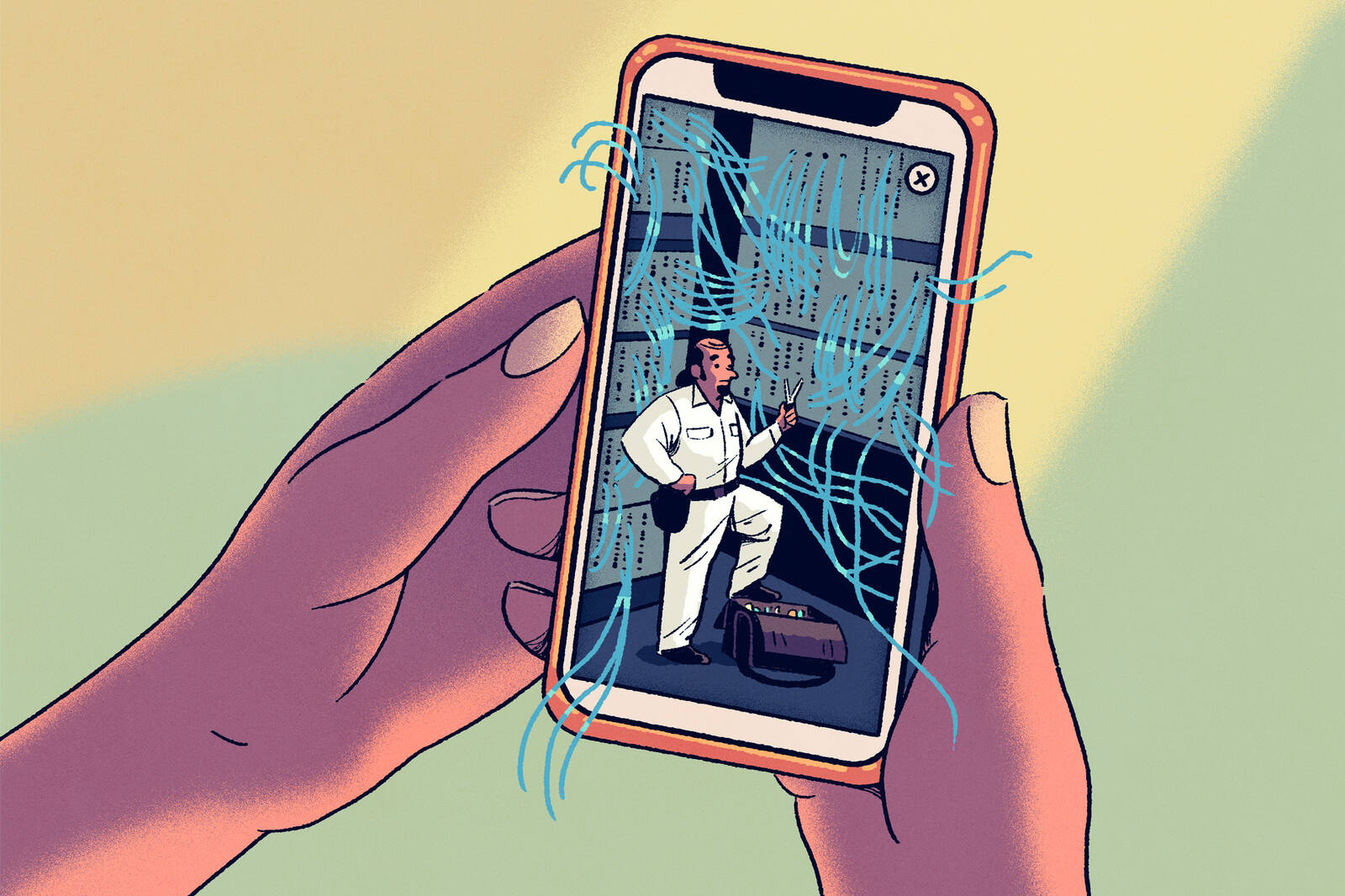
Michael Meier
Once a fashionable slogan for innovation, “move fast and break things” has fallen out of favor in the past decade. Tech companies have instead preferred “continuous experimentation.” However people choose to describe the concept, the goal is one and the same: to achieve speedy, data-driven iteration. The more successful experiments a firm runs—whether it’s testing the color of a button or the performance of an algorithm—the more opportunities it creates for new growth and efficiency.
But not all successful experiments are created equal. Some experiments lead to changes that seem positive in the short term (e.g., lead to more profit, customer retention, etc.), but actually smuggle new complexity into the workings of the company itself. This makes it harder to further improve the system and run future experiments, slowing down the very engine that makes innovation possible.
“The fundamental question is, What does it mean to say that something ‘worked’?” asks Sébastien Martin, an assistant professor of operations at Kellogg. “Right now it may be amazing for me and my customers, but long term, does it impact my ability to innovate?”
To investigate the hidden costs for experimentation-driven companies, Martin and his collaborators Yudi Huang (also from the Kellogg School) and Zhiwei Qin (a former principal scientist at Lyft) created a mathematical model that captures the complexity of making changes within a company. They discovered that the friction created by this complexity compounds over time, like interest on a debt. In other words, each successful change becomes increasingly harder to discover, requiring more-complex and time-consuming experiments—and these slowdowns often remain undetected.
However, the researchers also found that after a company passes a certain threshold of complexity, it’s no longer worthwhile to resist it. So despite having to deal with slower and more-infrequent experimentation, it actually pays to accrue more “complexity debt,” even as the returns on experimentation diminish. “The only solution in this case is often to rebuild from scratch rather than trying to prevent additional complexity,” Huang explains.
“The model is saying something very counterintuitive,” admits Martin. “It highlights an effect almost nobody talks about.”
Understanding this often-hidden effect of experimentation can be particularly valuable for tech companies that invest a lot of resources into experiments. “Tech companies have huge teams focused on experimentation; successful experiments are what get people promoted,” Martin says. “But saying whether something ‘works’ in the long term is a surprisingly hard task.”
The hidden costs of success
Martin experienced this difficulty firsthand. While working as a researcher at Lyft in 2020, he helped test a new reinforcement-learning algorithm for matching drivers with riders.
“It was very expensive to run these experiments, but definitely worth it,” he recalls. “It increased the revenue of drivers. It made customers happier. Almost all the metrics in our experimentation dashboard were green.” Lyft globally deployed the new algorithm in 2021. Everything worked out—or did it?
“I realized that when you make a complex change like this, it becomes harder for other teams [at the company] to innovate,” Martin says. “It also makes the process of experimentation itself harder and more costly.”
“The idea is that if you just keep implementing changes—following successful experimentation results blindly, all the time—there’s no limit to how bad [the negative impact of complexity debt] can get.”
—
Sébastien Martin
For one thing, having a sophisticated machine-learning algorithm that changes itself over time means that the next experimental idea becomes harder to find and more difficult to implement. “I have to anticipate what this crazy-smart algorithm will do in reaction to whatever I want to test,” Martin explains, “so it’s way harder to intuitively know if my idea is a good one.”
What’s more, complex system-wide experiments aren’t as simple as A/B testing the color of a button. Like a large rock dropped into a rushing stream, Lyft’s new algorithm might change the flow of the whole system in unpredictable ways—so the only way to test it was to turn it on for everyone to see what happens, then turn it back off and compare outcomes. These so-called “switchback experiments” had to be repeated many times, and over a longer period of time, in order to generate reliable results.
Martin sensed that the complexity debt from these experiments might subtly hamstring future innovation efforts, but he couldn’t be sure. “There is almost no way to measure them during the experimentation process,” he says. “I started to second-guess myself. And that led to an interesting mathematical exercise.”
Modeling trade-offs
Like technical debt, “complexity” within a company is hard to measure because it’s hard to define.
“It captures so many things—bureaucracy, software, depth,” Martin says. “For our purposes, complexity only has one meaning: the probability of running a successful experiment at your company on any given day is lower when your complexity is higher.”
Using this definition of complexity, Martin and his coauthors modeled how a company changes over time in response to continuous experimentation. They assessed this idealized company in terms of two key quantities. The first, called a utility rate, represents the main metrics the company tries to maximize through experimentation, such as profit or user engagement. The second term represents complexity: the higher the complexity, the more it drags down the rate of experimentation.
From there, the trade-offs are relatively simple. Over time, successful experiments increase the company’s utility rate—just like Lyft’s new algorithm led to an increase in revenue, engagement, and efficiency. At the same time, any change resulting from a successful experiment either increases its complexity or leaves it unchanged.
Setting traps
After analyzing the model’s behavior, the researchers found that complexity is indeed a real problem, manifesting in three distinctive patterns or “traps.”
The first trap is that the negative impact of complexity debt has no ceiling; it will simply grow and grow without ever plateauing. “The idea is that if you just keep implementing changes—following successful experimentation results blindly, all the time—there’s no limit to how bad it can get,” says Martin.
The second trap occurs when a company’s complexity debt becomes self-reinforcing. In the model, a company can make choices that keep that complexity from getting any worse. However, after a certain threshold of complexity is reached, even this approach becomes pointless: the optimal choice for a highly complex company is to continue experimenting and accruing more debt.
Why? Because managing complexity is costly in the short term and only beneficial in the long term.
“Public companies have investors who care about, at maximum, five-to-ten years from now,” Martin explains. “And when you’re a big company, and your complexity is high, improvements are rare. So you have a much stronger incentive to just get any improvement you can,” regardless of the long-term complexity costs.
But companies with low complexity (like startups) should be especially careful about keeping it at bay. These companies tend to care even more about short-term revenue and growth than do more-mature companies and are particularly susceptible to the third trap, where they feel the need to experiment as fast as possible. This is the dilemma that tech startups often face: because they have to demonstrate relatively rapid growth to their investors to survive the next round of funding, they tend to take up “greedy” experiments that increase complexity toward (and over) the threshold. And when they do start to grow, their complexity debt is already at that point.
“Startups are very different than post-IPO companies,” says Martin. “Any change you could make that seems to help you grow should be implemented—otherwise, you’ll die.”
No free lunch
So, is complexity—and its insidious effects on experimentation and innovation—simply inevitable, like death and taxes?
Perhaps, but Martin cautions against over-interpreting the behavior of just one mathematical model. “Our paper was intended to highlight an effect in the context of experimentation, which then allows people to start thinking about it more clearly,” he says. “When you’re trying to solve a problem, half of the game is to just be aware of it.”
Contrary to what many companies seem to believe, Martin adds, continuous experimentation is not a free ticket to continuous improvement. “Based on my own experience in the tech sphere, I think this idea would be very controversial,” he says.
But it doesn’t have to be. So-called “degradation experiments” act like switchback tests in reverse, measuring what happens when a seemingly positive change is temporarily reverted later. If there are no deleterious effects, maybe the change—and its resulting complexity—can be permanently reversed, says Martin, because “the system has evolved” and it’s no longer needed. And in other experiment-driven sectors like pharmaceutics, studying long-term outcomes—not just short-term effects—is the norm.
“We need a bit more of this thinking in the tech industry,” Martin says. “It puts the idea into people’s minds that changes may be temporary, because there might be a price to them—and that reverting, or at least reevaluating, is OK.”
John Pavlus is a writer and filmmaker focusing on science, technology, and design topics. He lives in Portland, Oregon.
Huang, Yudi, Sébastien Martin, and Zhiwei (Tony) Qin. 2025. “The Trap of Complexity in Experimentation.” Working paper.