Organizations Policy Aug 16, 2023
Social-Media Algorithms Have Hijacked “Social Learning”
We make sense of the world by observing and mimicking others, but digital platforms throw that process into turmoil. Can anything be done?
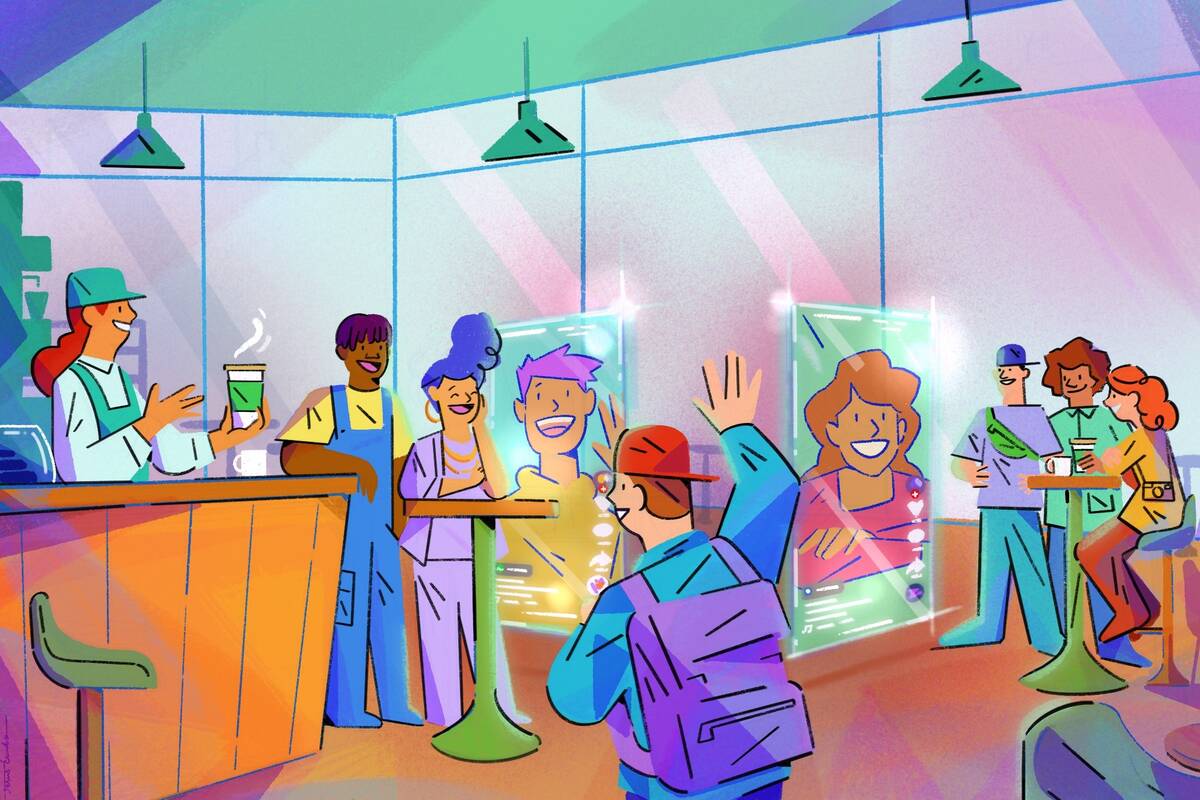
Jesús Escudero
In middle school, how did you learn what clothes and bands were considered cool? It’s possible someone told you, but there’s a good chance you picked it up simply by watching what your classmates were listening to and wearing. This process, called social learning, is one of the most important ways we make sense of the world around us.
“Humans are natural social learners. We are constantly scanning the environment to figure out what other people are doing and what we can learn from that,” says William Brady, an assistant professor of management and organizations at Kellogg. “Social learning happens whenever we observe people, get feedback from them, mimic them, and incorporate this information into our understanding of norms.”
Social media represents a new frontier for this type of learning. What happens when this all-important observing and mimicking of others is mediated by algorithms controlled by tech companies whose goals are to keep people’s attention on platforms?
All kinds of trouble, according to Brady, Joshua Conrad Jackson of the University of Chicago, Björn Lindström of the Karolinska Institutet, and M.J. Crockett of Princeton. In a new paper, they present a framework that describes the perils of social learning in the digital age.
The researchers argue that the way platform algorithms filter content interferes with the strategies people typically use for social learning, leading to misperceptions about the world and facilitating the spread of misinformation and extreme views. Fortunately, Brady also believes adjustments to the algorithms would lessen these harms, while still offering engaging material for users.
Why we lean on PRIME information in social learning
When we’re in the thick of social learning, we use shortcuts to determine what information is most important. After all, we can’t pay attention to everything.
So instead, previous research suggests, we direct our attention toward information that Brady dubs “PRIME”: prestigious, in-group, moral, or emotional. Prestigious information comes from someone who is considered successful, in-group information comes from a peer, moral information gets at whether people are behaving in line with shared ethical norms, and emotional information is emotionally charged (and often negative).
There are some very good reasons why humans are biased toward attending to PRIME information. For instance, “it can be useful to have a bias toward information learned from successful individuals. They have some kind of knowledge that has helped them succeed in their environment,” explains Brady. Biases toward ingroup information are useful for helping people navigate their specific social environment and, more broadly, can facilitate cooperation. Biases toward moral and emotional information, respectively, can help stigmatize amoral behavior and identity social threats.
“Humans are natural social learners. We are constantly scanning the environment to figure out what other people are doing and what we can learn from that.”
—
William Brady
Critically, however, PRIME information is most useful when it is both rare and diagnostic (meaning the person who seems successful actually is successful, or the behavior characterized as unethical actually is unethical).
This helps explain why the usefulness of PRIME information can break down in online environments, the researchers argue.
How so? In short, because of algorithmic amplification, we are flooded with PRIME information that is neither rare nor particularly diagnostic.
When PRIME information breaks down
Social-media algorithms are designed to maximize engagement: clicks, likes, time spent on the platform, and so on. And because our brains are biased to see PRIME information as important—and therefore engaging—algorithms have learned over time to serve us a whole lot of it. As a result, there’s an incentive for users to post in ways that appeal to our taste for prestigious, in-group, moral, and emotional content.
Often, this results in outright fakery. Take a picture in what appears to be a private jet, and you’ll look like you’ve attained prestige and wealth (even if it’s just a $64-an-hour photo studio).
Online, then, prestige and success are not as tightly coupled as they are in most real-world environments. And when algorithms amplify social-media influencers who have “faked” success with a highly polished presentation, and other users take lessons from their words or actions, the functionality of the bias toward prestigious sources of information has broken down.
This same breakdown can happen with other types of PRIME information, too. The social-learning bias toward in-group information has historically fostered cooperation and understanding within a community—to get along, it helps to have a shared set of norms.
But online, it can play a more divisive role in how people perceive social norms and politics. It’s easy for in-group information to foster groupthink and, eventually, extremism. And when social media users see extreme views regularly and accompanied by lots of likes, they may begin to believe the viewpoint is more common than it is.
“Take the right context and the right people, add algorithmic amplification, and it can skew social learning in ways that make extreme views seem more legitimate and widely held,” Brady says.
Brady sees the January 6 insurrection as, well, a PRIME example. “How does a fringe right-wing view gain legitimacy and get a critical mass of people to organize and storm the Capitol?” Brady asks. “People would not have seen these views if they were not being amplified by algorithms, and that happens because they get engagement. Algorithms put fringe views in the public discourse and allow people to organize around them.”
Online, PRIME information can also lead people to believe the country is more polarized than it is. Most partisans vastly overestimate how far their views are from those on the other side of the political spectrum, and social-media interactions are one source of this misunderstanding.
When platforms expose users to information about their political out-group—those who do not share their political leanings—the posts they present are often extreme and colored by commentary from their political in-group. And that commentary is often negative, moralized, and emotional.
“That is exactly the type of information that algorithms amplify. People see a skewed portrayal of the other side,” says Brady.
How “bounded diversification” could change social media for the better
So, engagement-based algorithms and PRIME information make for a conflict-laden, unhelpful combination. Can the problem be combated?
One way would be for platforms to show social-media users a more-diverse range of views. However, that approach could have unintended consequences, such as showing extreme views to moderate people—not necessarily an improvement.
Instead, Brady and his coauthors propose two alternative solutions. One is to increase the transparency of social-media algorithms. Simply telling users why they are seeing a given post—because a close friend shared it or because the platform felt it would be engaging, for example—would help users understand how the technology works and think more deeply about what they’re consuming online.
The researchers call the other solution “bounded diversification.” This approach involves tweaking algorithms to limit the amount of PRIME information users see.
Today, algorithms rank the relevance of content and show users the posts most likely to drive engagement. As we’ve seen, this introduces too much PRIME information into the mix. Bounded diversification would introduce a penalty for PRIME information, so that algorithms rank this type of content lower and show it to users less often.
The non-PRIME information left in the mix would still be content the algorithm identified as likely to engage users. Depending on the user, this could be funny memes, historical photography, or cute puppy videos—content that would still grab attention but be less likely to inflame.
Brady sees this change as one platforms could stomach. “At the end of the day, we know social-media companies want people to be engaged because that is how they make money. They need to keep their platforms going,” he says. “So, we propose this approach because it would still give people content that they find interesting but wouldn’t be so dominated by PRIME information.”
Ty Burke is a freelance finance and economics writer.
William J. Brady, Joshua Conrad Jackson, Björn Lindström, and M.J. Crockett. 2023. “Algorithm-Mediated Social Learning in Online Social Networks.” Trends in Cognitive Sciences.