Finance & Accounting Policy Feb 23, 2024
The Dos and Don’ts of Regulating AI
How can governments capitalize on AI’s benefits while minimizing its dangers? New research examines several policies—and identifies a promising approach.
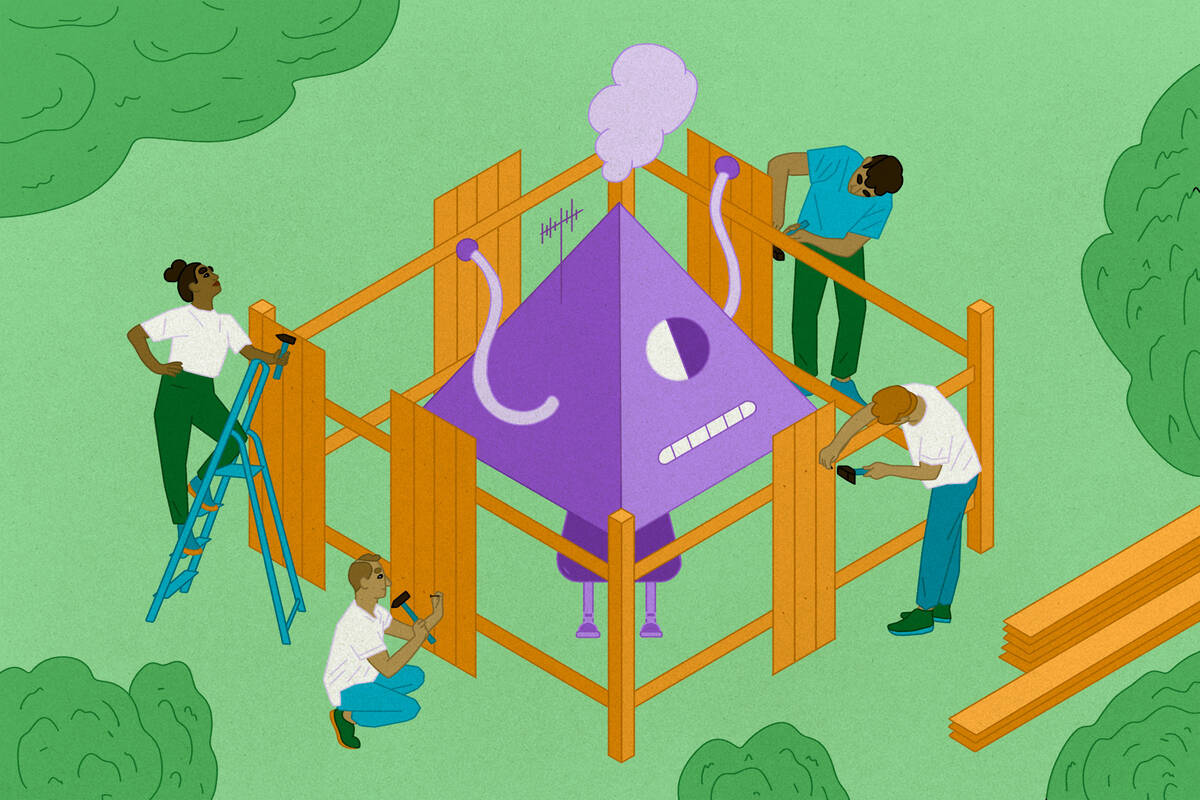
Lisa Röper
Policy wonks have had their eye on neural-network algorithms—colloquially known as “artificial intelligence,” or AI—since at least 2016, when Google’s Deep Mind famously used the technology to beat the world’s best human player at the ancient game of Go.
In 2023, soon after OpenAI released its powerful ChatGPT app to the public, AI regulation went from niche concern to front-page news. The European Union hastily revised its AI Act to account for the sudden appearance of software that could generate college essays, computer code, and misinformation with equal effortlessness. OpenAI CEO Sam Altman beseeched Congress to rein in his industry ahead of the 2024 presidential election. And the Biden administration issued an executive order requiring AI developers to share “safety test results and other critical information with the U.S. government.”
While observing this flurry of regulatory activity, Kellogg finance professor Sergio Rebelo and his coauthors, João Guerreiro of UCLA and Pedro Teles of Portuguese Catholic University, noted that the new frameworks each “tended to emphasize one solution.” For example, that solution might revolve around banning certain high-risk AI applications outright (as in the EU’s revised AI Act); requiring tech companies to safety-test their AI software and report the results to the government (as Biden’s executive order called for); or holding AI developers legally liable for harms caused by their algorithms (as per the AI Liability Directive, a proposed law in the EU). The researchers wondered which, if any, of these approaches was likely to be successful.
“We wanted to try to think about these concerns in a systematic way by creating an economic model that would capture what the main issues are while screening out the details of how a specific algorithm works,” says Rebelo. In other words, by using mathematics to intentionally simplify reality, the researchers wanted to bring the key problems facing AI regulators into clearer focus. The reason for this simplification, he adds, is that the specific social effects of any particular AI algorithm are simply too uncertain to anticipate in any realistic detail. “We really are in a brave new world,” he says. “We don’t really know what our algorithms might bring.”
By building this inherent uncertainty about AI into their model, the researchers were able to clarify—in broad strokes—which regulatory approaches are most likely to work. The bad news: according to their model, none of the approaches currently being considered by the U.S. and EU fits the bill. At least, not alone. But in a certain combination? “That can get close to an optimal solution,” Rebelo says.
Setting up the machine
The “optimal solution” the researchers identified represents an ideal balance between AI’s potential risks to society (like destabilizing democracy through rampant disinformation) and its potential rewards (such as increasing economic productivity).
To find this balance—and which policy levers might be pulled to encourage it—the researchers’ model was designed to represent the regulation of any hypothetical new AI algorithm, regardless of its technical details.
First, Rebelo and his collaborators captured the intrinsic uncertainty about AI’s social outcomes with a variable that reflects how severe any unintended negative consequences of AI might be—what economists call “externalities.” For example, a large externality associated with AI might be the disruption of elections or financial markets. A smaller externality might be an increase in student plagiarism due to the use of chatbots.
Second, the researchers introduced a variable called novelty. This variable allowed their mathematical model to function without having to account for the technical details behind any particular AI algorithm—whether known or yet to be invented. Instead, novelty simply describes how much an algorithm differs from the existing state of the art.
“We really are in a brave new world. We don’t really know what our algorithms might bring.”
—
Sergio Rebelo
“Transformers, the architecture used by ChatGPT, are a significant departure from prior algorithms used in natural language processing, Rebelo says, so his model would assign them a high novelty value. In contrast, a more incremental improvement to AI’s status quo—like improving a smartphone’s facial-recognition software—would warrant a low novelty value.
Importantly, the model links uncertainty to novelty. “The bigger the novelty, the more uncertainty there is” about possible externalities, Rebelo explains. “That’s really key to the analysis. Imagine explorers in the 15th century: the further you sail from your homeland, the more uncertainty there is about potential dangers. That’s how we were thinking about how to model the concerns about regulating AI.”
Finally, the model considers social welfare, with a variable that aggregates all the individual benefits and costs associated with producing and using AI. For instance, AI developers have an incentive to develop software that will generate profits and avoid liability, while customers will seek out software that is personally useful and cost-effective.
Both developers and customers will seek to maximize their own welfare, sometimes at each other’s expense. But when their incentives are aligned, their combined welfare—that is, social welfare—rises. A social “optimum” balances trade-offs between the expected social welfare of an innovation and its associated risks.
With this mathematical model established, Rebelo and his colleagues could then apply different AI-regulation scenarios and “turn the crank” to observe how uncertainty, novelty, and welfare interacted.
“What we found,” Rebelo says, “is that none of these [scenarios] in isolation will deliver it.”
Dos and don’ts
One approach to regulating AI is to classify algorithms into risk tiers and ban the use of any applications deemed too dangerous—an approach currently favored by the European Union. The upside of this approach is obvious: “you just completely eliminate a class of risk,” says Rebelo.
The downside, though, is that this approach doesn’t account for unpredictable externalities—and AI is nothing if not unpredictable. This inherent uncertainty means that even algorithms initially classified as low-risk—especially very novel ones—have a chance of causing “really bad consequences,” Rebelo says. A ban-based regulatory regime “might be useful, but it’s not enough” to achieve the optimal level of social welfare.
Another way of regulating AI is to mandate beta testing of all algorithms to evaluate their safety in controlled settings before releasing them to the public. In Rebelo’s model, this approach can reduce overall social welfare by creating too much red tape around “trivial deviations” from the status quo, he says. “It would be like going through a whole new clinical trial because you changed the color of an aspirin pill.”
So if mandatory testing and risk-based bans won’t work on their own, what will (at least, according to the model)? “We found that a combination of beta testing and limited liability will yield an optimal solution,” says Rebelo.
In this scenario, the costs of mandatory safety testing are reduced by allowing room for discretion around an algorithm’s novelty. “The regulator decides whether a certain algorithm should be tested or not, and you can exempt some that are very close to the status quo,” Rebelo says. “It’s much like how companies do research on chemicals or virology. They submit a proposal and if it looks fairly low-risk, you don’t have to do testing.”
However, Rebelo adds that AI developers would still have an incentive to “swing for the fences” in terms of novelty. “We see this in real life all the time,” he says. “Obviously, being the first to release a novel algorithm can generate a lot of profits. The thought is, ‘Sure, there may be some downside for society, but we can worry about that later.’”
Adding limited liability to the regulatory equation puts a check on this impulse. “If I release an algorithm to society and then something really bad happens, and I’m liable for it, then I’m going to withdraw the algorithm from circulation,” Rebelo says. The threat of this outcome also encourages AI developers to self-regulate how far they push the envelope with their algorithms in the first place. “It’s a nuanced policy,” he says, “but it corrects the misalignment between private and social incentives.”
Reality check
Of course, actually implementing effective AI regulation in the real world is no small feat. Even if OpenAI wanted to “withdraw” ChatGPT from the market to mitigate the many externalities it’s been responsible for since 2023, the transformer algorithm powering it is already embedded into so many other open-source applications that putting the genie back into the bottle is all but impossible.
Furthermore, Rebelo and his colleagues note that their regulatory model only works within a single country—while software has a global reach.
Still, Rebelo says, the model does suggest a general direction for thinking about how to regulate AI effectively. “It would be very similar to how drug approval works,” he says. “What we’re trying to describe is, how would we march towards a consistent regulatory framework that would better align the incentives of developers and incentives to society? That’s the value of writing down models, because otherwise, there’s no basis for discussion.”
John Pavlus is a writer and filmmaker focusing on science, technology, and design topics. He lives in Portland, Oregon.
Guerreiro, João, Sergio Rebelo, and Pedro Teles. 2024. “Regulating Artificial Intelligence.” Working paper.