Social Impact Nov 1, 2023
The Big Trade-off at the Heart of Generative AI
Tools like ChatGPT can improve efficiency at the individual level—but could lead to large societal problems.
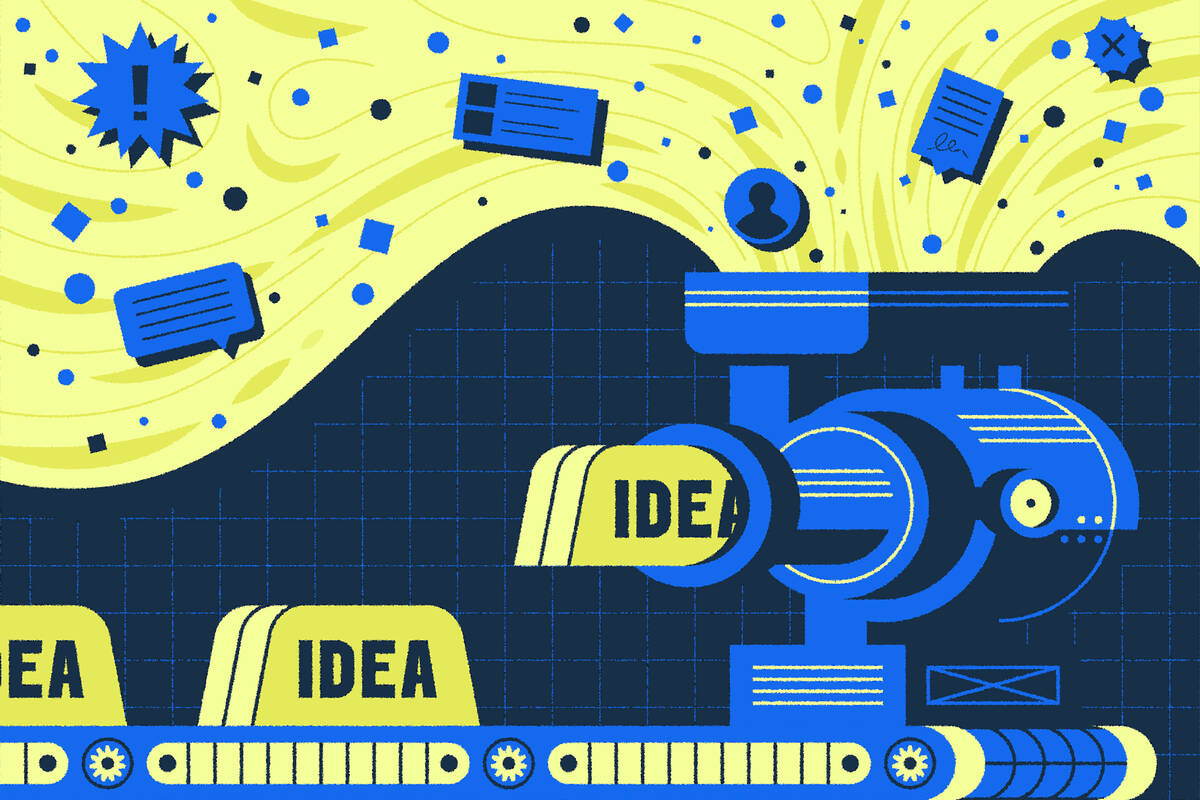
Riley Mann
If you’ve started to incorporate generative AI tools like ChatGPT into your workflow, you’ve probably noticed something pretty striking: while AI can help you write or code or illustrate more efficiently, it can also take a lot of work to shape the output into something similar to what you would have created yourself.
Generative AI, then, presents users with a fundamental trade-off: to maximize its efficiency benefits, you need to sacrifice some of the output’s “fidelity”—that is, how faithfully or exactly it adheres to your unique style or perspective.
“If the whole point is to work faster and to increase productivity, then it has to be that somewhere you’re letting go of something for the sake of speed,” says Sébastien Martin, an assistant professor of operations at Kellogg.
For individuals, this trade-off can be aggravating, but at least it is straightforward. Either accept the AI-generated output as “good enough” or put more time into personalizing it, perhaps by providing more information upfront, tweaking the prompt, or editing the output afterwards. Individuals who possess a particularly distinctive style or perspective may even decide that personalizing the AI is more trouble than it’s worth and abandon the tool altogether.
But what happens when everyone starts to use these tools? Does the speed–fidelity trade-off have broader societal consequences, both in the short-term and over time?
In new research with his colleagues Francisco Castro and Jian Gao of UCLA, Martin finds that using AI to create content will increase the homogeneity of what we collectively produce, even if we try to personalize the output. Further, this content will also inherit any biases the AI may have acquired during its training process. In other words, the tastes and biases of a few AI company workers may ultimately permeate throughout society. The research also finds that these effects will be compounded as AI-generated output is used to train the next generation of AI.
On a more positive note, however, the study suggests that creating interactive AI tools that encourage user input and facilitate manual edits can prevent the worst of these outcomes.
Opportunities and risks
Martin is well-acquainted with the speed–fidelity trade-off that is inherent to generative AI. He is, after all, a native French speaker who regularly relies on Grammarly to improve his written English. “I save a lot of time!” he says.
Still, Martin acknowledges that using the tool inevitably shapes the articles he writes. There’s nothing particularly nefarious about this: idiosyncratic preferences around punctuation, word choice, and sentence structure abound. “What Grammarly will write will not be exactly what I would have written,” he says. “There are different ways to write the same thing. Sometimes it’s just a matter of taste.”
But other times, differences are more meaningful. Whether we describe an event as a “protest” or a “riot,” or draft a news article from a center-right versus center-left perspective, can meaningfully shape an audience’s impressions about what happened. And more broadly, what happens over time when everyone’s collective taste is influenced by the same algorithms?
Over time, “any AI bias can actually turn into a societal bias.”
—
Sébastien Martin
To find out, Martin, Castro, and Gao built a mathematical model to simulate the consequences of an entire society using the same AI tools.
In their model, users with a range of different preferences use AI to work on a given task and can choose to personalize the output as much as they want. This personalization is represented as an exchange of information about each user’s preferences. Users decide how much effort to expend, depending on their distinct situation: sharing more information means the AI will do a better job of capturing unique preferences, but it also takes more work. Sharing less information is quicker and easier, but it produces a more-generic result. Even the best AI is not able to guess the true preferences of a user if that user shares limited information, but it can make an educated guess as it learns the variation of preferences in the population at large during its training.
How would individual users decide to use these tools, the researchers wondered, and what would their choices mean in aggregate?
Inspired by algorithms
The model confirmed that, for users with the most common or middle-of-the-road preferences, the optimal decision was to accept the AI output as is. Users with less-common preferences, however, would be incentivized to share additional information with the AI or edit the output themselves to steer it away from the default. Meanwhile, for users with fringe preferences, AI was not a time-saver at all: these users were better off just creating the content themselves.
The model also found that AI-generated content is always more homogenous than user-generated content. This is true at the individual level, where the benefits of AI come from substituting some amount of our own eclectic preferences for more-popular ones. But it is also true at the population level. The range of preferences expressed in the AI-generated content was less variable than the range of preferences in the population—an effect that was amplified because those users with fringe tastes simply didn’t use the tool at all.
Moreover, the uniformity compounds over time, as AI-generated content is then used to train the next generation of AI. This creates what the researchers call a homogenization “death spiral.” The new AI is trained on more homogenized data and therefore is more likely to create homogenized content. Users then need more time and effort to tweak the AI output to fit their preferences, which they may not be willing to do, leading to even more homogenization.
Another problem with AI—bias—will also compound over time, the model suggests. Given that most AI is created and trained by a limited number of people (a typical approach is RLHF, or Reinforcement Learning with Human Feedback), it is nearly inevitable that some bias will slip into the initial AI outputs. Users can fix this bias with some effort, but if the bias is small enough, it may not be worth it for many—or they may not even notice.
But this understandable individual behavior compounds if we all act similarly. Over time, “any AI bias can actually turn into a societal bias,” says Martin.
This allows AI companies to have a huge influence on societal outputs, even if users try their best to limit it.
A way forward
There are ways to mitigate the societal problems associated with AI, the researchers find. One of the most promising is to get more people interacting with it and editing the work themselves. Homogeneity and bias will not run rampant so long as the model’s output is able to reflect users’ actual preferences—which means users need to actually make those preferences clear.
In practice, this might mean an AI asking users a few questions before generating a result, to get a better sense of their unique style or perspective. Or it might mean providing multiple outputs.
“Instead of giving you one version, try to give you two very contrasted versions to allow you to choose between them,” suggests Martin.
He acknowledges that these suggestions will slow users down in the short term—making the technology slightly less useful. But in the long term, this strategy “would be a very good thing for sure”—both for users and for the AI tools.
Martin remains largely optimistic about the role that generative AI can play in content creation—so long as it continues to reflect the full range of human preferences. Indeed, making creation more accessible to a plethora of new writers, artists, or coders could even have benefits.
“AI can also bring more people into something that they could not do before,” he says, “which could potentially add a little bit of diversity.”
Jessica Love is editor in chief of Kellogg Insight.
Castro, Francisco, Jian Gao, and Sébastien Martin. 2023. "Human–AI Interactions and Societal Pitfalls." Available at https://doi.org/10.48550/arXiv.2309.10448