Healthcare Feb 5, 2024
What Happens When We Give Doctors an AI Assistant?
Machine-learning systems can improve physicians’ accuracy at diagnosing dermatological diseases. But even with AI assistance, physicians struggle to close the accuracy gap between light- and dark-skinned patients.
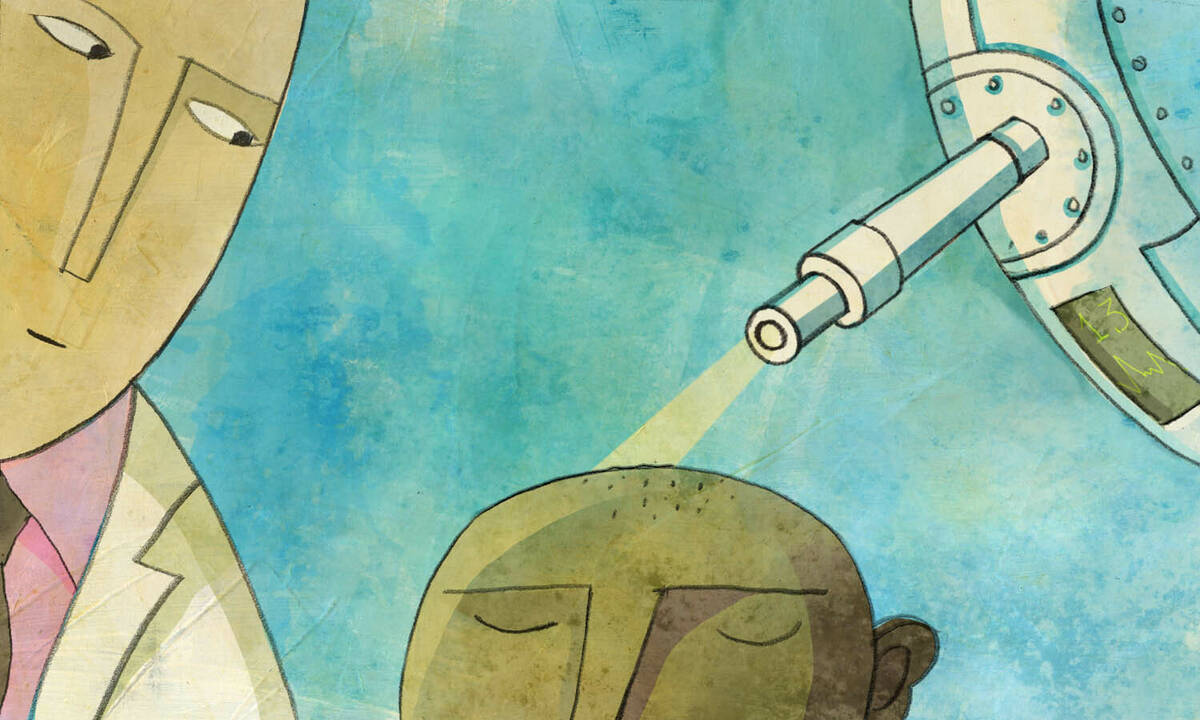
Yevgenia Nayberg
Like many fields, medicine is still figuring out how it will make the most effective use of artificial intelligence. While robots are unlikely to replace doctors anytime soon, it’s easy to imagine a future in which the two work together.
These physician–machine partnerships hold particular promise for dermatology, a specialty in which diagnosis often comes down to recognizing the visual characteristics of a disease—something that deep-learning systems (DLSs) can be trained to do with great precision.
There’s even hope that machine learning could help address a known problem in the field: only 10 percent of images in dermatology textbooks depict patients with darker skin, meaning that physicians may be unfamiliar with the different ways diseases can present across skin tones.
New research from Matt Groh, an assistant professor of management and organizations at the Kellogg School, puts the issue of machine-aided dermatology to the test by seeing how suggestions from deep-learning systems affected physicians’ photo-based diagnoses. The research was coauthored by dermatologists Omar Badri, Roxana Daneshjou, and Arash Koochek; Caleb Harris, P. Murali Doraiswamy, and Rosalind Picard of the MIT Media Lab; and Luis R. Soenksen of the Wyss Institute for Bioinspired Engineering at Harvard.
“The question was, well, does a dermatologist plus AI assistance do better or not?” Groh explains. The researchers looked not only at overall accuracy levels, but also fairness—whether accuracy levels increased evenly across images of lighter and darker skin.
The results were mixed. Assistance from even an imperfect deep-learning system increased dermatologists’ and general practitioners’ diagnostic accuracy by 33 percent and 69 percent, respectively. However, the results also showed that, among general practitioners, the DLS exacerbated disparities in accuracy across light and dark skin. In other words, generalists supported by AI got much better at making correct diagnoses in light skin but only slightly better in darker skin.
To Groh, the results suggest that machine learning in medicine is powerful but not a magic bullet. “AI in health care can really help improve things,” he says. “But it matters how we design it—the interface in which the AI is deployed to the humans, how the AI performs on diverse people, and how the practitioners perform on diverse people. It’s not just about AI. It’s about us.”
Giving doctors a robotic consult
For the study, Groh and his colleagues curated a set of 364 images representing different skin conditions in patients with a variety of skin tones. The researchers made sure to include conditions that look different in light and dark skin—Lyme disease, for example, generally appears as a red or pink bullseye rash in people with lighter skin, but may present as brown, black, purple, or even off-white in people with darker skin.
They used two different deep-learning systems in the experiment. The first, which had been trained on images without any interference from the researchers, had an overall accuracy rate of 47 percent and was designed to mimic today’s work-in-progress machine-learning dermatology tools. The second had been enhanced by the researchers to achieve 84 percent accuracy and was an attempt to simulate the more precise tools that will likely become available to doctors in the future.
The researchers used Sermo, a networking site for medical professionals, to recruit more than 1,100 physicians for the study. Participants included dermatologists and dermatology residents, as well as general practitioners and other medical specialists.
Including both specialists and generalists was important, Groh says, given that “general practitioners often see skin disease, because dermatologists are hard to book. A lot of times, you might talk to a general practitioner before you see a specialist.”
“AI in health care can really help improve things. But it matters how we design it.”
—
Matt Groh
The participating physicians went to a website where they answered a series of questions about their experience diagnosing skin conditions in patients with different skin tones. Then, they were presented with ten different photos of skin conditions and asked to give their top three diagnostic guesses for each, mimicking the differential-diagnosis process doctors use in their real practices. If the doctors guessed incorrectly, they saw a proposed diagnosis from the deep-learning system and were given the opportunity to update or keep their own diagnosis.
Participants were randomly assigned to receive recommendations from either the less-accurate control DLS or the more-accurate treatment DLS. (While they’d been told at the beginning of the study that the DLS was not perfectly accurate, the doctors did not know overall accuracy rates for either system.)
The researchers also varied the way in which they prompted doctors to update their diagnosis. Half were shown a “keep my differential” button as the first of three options, while the other half saw “update my top prediction” first—a more forceful prompting to accept the AI’s suggestion.
Understanding diagnostic accuracy and fairness
Across all skin conditions and skin tones in the experiment, dermatologists, dermatology residents, generalists, and other physicians were 38 percent, 36 percent, 19 percent, and 18 percent accurate, respectively, meaning that they included the correct diagnosis among their three guesses. Top-1 accuracy—the accuracy of the diagnosis listed first—was 27 percent, 24 percent, 14 percent, and 13 percent, respectively.
While those numbers might seem low, Groh says it’s important to remember the experiment was very constrained—much more so than teledermatology would typically be in the real world. “It’s a hard task when you only have one image, no clinical history, no photos in other lighting conditions,” he explains.
Doctors’ diagnostic accuracy decreased even further when the researchers narrowed their analysis to darker skin. Among generalists, having little experience with darker-skinned patients had a particularly deleterious effect: primary-care providers who reported seeing mostly or all white patients were 7 percentage points less accurate on dark than light skin.
And how did the DLS change things?
Even the less-accurate control system meaningfully boosted accuracy: top-1 accuracy increased by 33 percent among dermatologists and dermatology residents and 69 percent among generalists. Not surprisingly, the more-accurate treatment DLS raised these figures even further.
“Ultimately, they’re making better decisions while not making many mistakes,” Groh says—meaning doctors aren’t accepting inaccurate suggestions from the DLS.
Among dermatologists and dermatology residents, DLS support increased accuracy relatively evenly across skin tones. However, the same was not true for generalists—their accuracy increased more in light skin tones than dark ones.
Interestingly, how doctors were prompted to adjust their diagnoses in response to DLS feedback had a meaningful effect on accuracy. When doctors were shown the “update my top prediction” first on the list as compared with last, their top-1 accuracy increased significantly.
These results suggest the critical importance of design. “These little details,” Groh says, “can lead to big differences.”
The right kind of physician–machine partnership
Groh says another important takeaway from the research is how difficult it is to diagnose skin conditions from photos alone. The relatively low overall accuracy rates provide “some sense of how much information is in an image,” he says. “It’s really imperfect.”
The fallibility of images means that the best AI-supported dermatology might look different from how we currently imagine it. Until now, many doctors and computer scientists have assumed the optimal approach would be to teach the system to produce a single diagnosis from an image. But perhaps, Groh says, it would be more helpful to train a DLS to generate lists of possible diagnoses—or even to generate descriptions of the skin condition (such as its size, shape, color, and texture) that could guide doctors in their diagnoses.
In the end, the research shows that DLSs and doctors are more powerful together than they are alone. “It’s all about augmenting humans,” Groh says. “We’re not trying to automate anyone away. We’re actually trying to understand how augmentation might work, when and where it will be useful, and how we should appropriately design augmentation.”
Susie Allen is the senior research editor of Kellogg Insight.
Groh, Matthew, Omar Badri, Roxana Daneshjou, Arash Koochek, Caleb Harris, Luis R. Soenksen, P. Murali Doraiswamy, and Rosalind Picard. 2024. “Deep Learning-Aided Decision Support for Diagnosis of Skin Disease Across Skin Tones.” Nature Medicine.