Economics Oct 29, 2020
What Is “Auction Theory,” and What Kinds of Questions Can It Answer?
The recent Nobel put the field of auction theory in the spotlight. An economist explains how it works, using his own research as a guide.
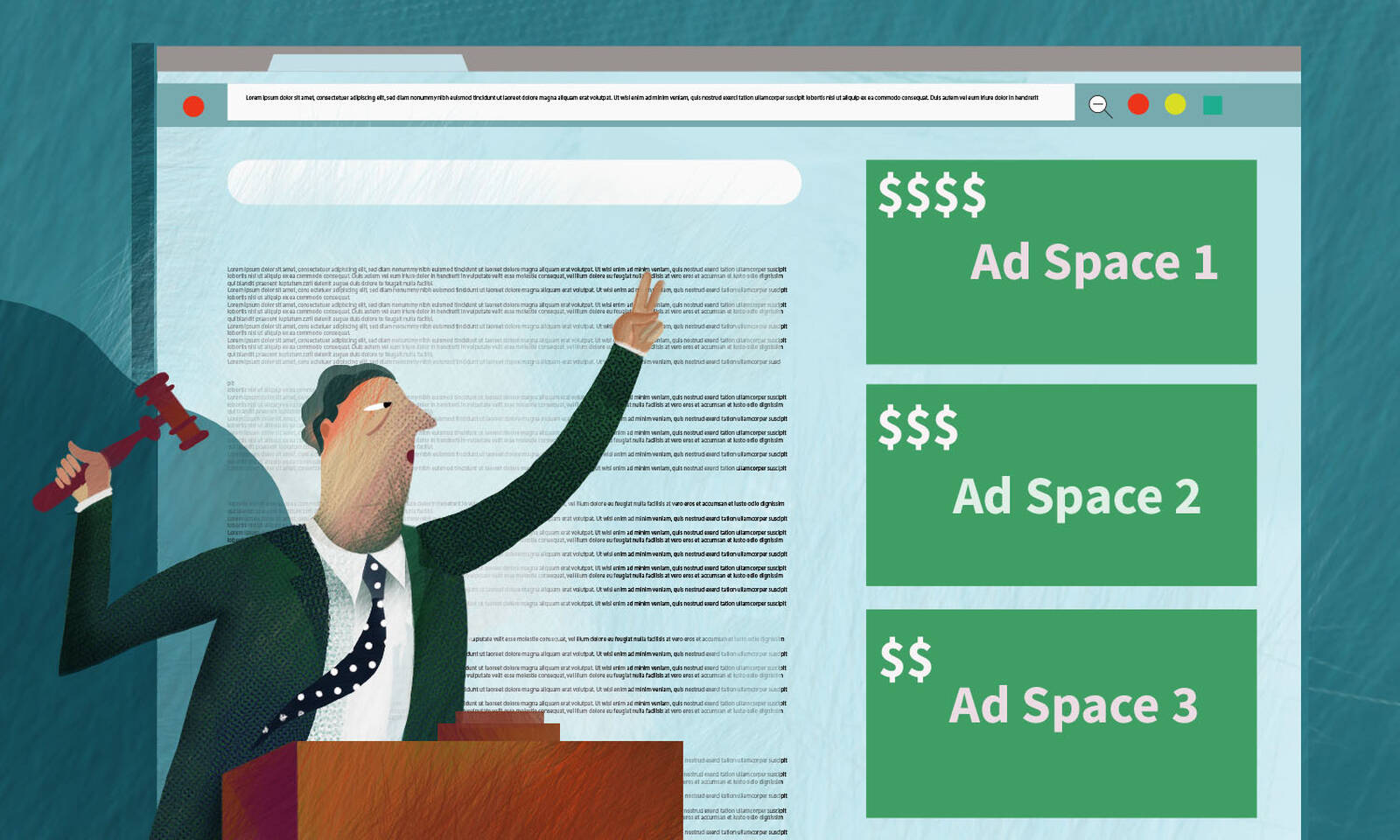
Yevgenia Nayberg
Auction theory studies different auction formats and attempts to predict how people will behave in them. The field is having its moment in the spotlight.
The attention stems from the recent awarding of the Nobel in economics to two pioneers of auction theory research, Paul Milgrom and Robert Wilson, both at Stanford University. (Milgrom is a former Kellogg School professor.) Their work is both theoretical and practical: in the 1990s the Federal Communications Commission used their research to create a new way of auctioning off radio frequencies, resulting in billions of dollars in sales.
Kellogg’s Joshua Mollner started collaborating with Milgrom while Mollner was a Ph.D. student at Stanford. The two have a new paper out on auction theory. Kellogg Insight talked with Mollner, an associate professor of managerial economics and decision sciences, about the research and the Nobel announcement.
KELLOGG INSIGHT: What did you set out to learn in your new paper?
We were motivated by the observation that in certain situations, existing theory doesn’t make very sharp predictions about how people will behave. We had an intuition for how we could develop a new theory that would sharpen those predictions.
The gold standard for making predictions about how people will play an economic game—for example, an auction—is something that’s called Nash equilibrium. What Nash equilibrium says, basically, is that everyone has to be using a strategy that allows them to do as well as they can provided that their opponents play as they’re supposed to be playing.
But in some settings, and particularly some auctions, there’s a problem because sometimes there can be many Nash equilibria. In other words, Nash equilibrium predicts many possible outcomes. And that might be dissatisfying because you might want just a single prediction or at least a small set of predictions.
In response, economists have developed rules, called “equilibrium refinements,” that allow you to eliminate some of these less plausible Nash equilibria so that you end up with a smaller set of predictions.
That’s where our paper fits in. We propose a new refinement, which we call “extended proper equilibrium.”
KI: Your refinement looks at the issue of “trembles,” meaning a player may do something unexpected—perhaps unintentionally, as if their hand trembled. Can you explain how that factors in?
In some games, many of the strategies I could adopt might be “tied” in the sense that each allows me to do as well as I can, given what I expect my opponents to do.
“When you do a search query on Google or really any other search engine, the ads that you see on the top or on the side are the result of an auction that’s taking place behind the scenes.”
— Joshua Mollner
But should I really be equally happy with any of those strategies? Maybe I should break that tie by thinking about the possibility that the other players don’t play as I expect them to play. Maybe with some small probability, another player trembles and does something that they weren’t supposed to do.
So, supposing that other players do tremble, what types of trembles should I expect? And that’s where our refinement comes in.
KI: Can you give an example of what this would look like?
The simplest example would be one where I’m playing a game against two opponents. Suppose that if I expect opponent #1 to tremble, then I prefer option A, whereas if I expect opponent #2 to tremble, then I prefer option B. To figure out what my best option is, what I really need to know is who’s more likely to tremble, opponent #1 or opponent #2?
It turns out that most previous refinements in the literature have basically nothing to say on that question. Our contribution is to develop a new refinement that does have something to say in some situations.
KI: What did you draw on for your idea for a new refinement?
We start with a previous equilibrium refinement from the literature. It was developed in the ’80s by Roger Myerson, who is also a Nobel laureate (and former Kellogg faculty member). He calls his refinement “proper equilibrium.” That concept has something to say about within-player comparisons of trembling: about whether a single opponent is more likely to make one type of mistake or another type of mistake. We find a way to extend that logic to across-player comparisons. That’s why we call our refinement “extended proper equilibrium.” That then allows us to make sharper predictions than the original proper equilibrium does.
KI: How does this apply to real-life auctions?
Let me give you one example. When you do a search query on Google or really any other search engine, the ads that you see on the top or on the side are the result of an auction that’s taking place behind the scenes. The most commonly used auction format there is called the “generalized second-price auction.”
Think about multiple ad positions on the page and multiple bidders. The ad position at the top of the page is the most valuable because it’s the most visible, and so it should attract the most clicks. As we go further down the page, ads are going to attract fewer clicks. Each bidder is going to submit the maximum amount that they’re willing to pay per click.
The top ad position goes to the highest bidder, but their per-click payment is not their own bid. It’s the bid of the second-highest bidder. And then the second ad position goes to the second-highest bidder, whose per-click payment is the third-highest bid, and so on.
KI: Why are they not paying their own bid?
In the olden days of search advertising, that was exactly what happened. But there was a problem with it. Let’s say I’m the highest bidder and you’re the second highest bidder, and I’m bidding $10 more than you are. If I’m paying my own bid, I have an incentive then to try to reduce my bid until I’m just barely above where you were. But once that happens, you might say, “Well, now I can outbid Josh and get that first position for very cheap.” At that point, we might keep outbidding each other until the first position becomes so expensive that you decide you’d be better off winning only the second position. So you might drop your bid back down to $10 below mine, starting the cycle all over again.
Search engines were seeing this cycling behavior, where bidding behavior wasn’t settling down. To eliminate it, they went to this design where now I can feel free to bid $10 higher than you, because whatever happens, I get the same outcome as if I were bidding just a single cent higher than you.
Google came up with this system, and now it’s the standard in the industry. That’s why economists are interested, because it’s economically important.
KI: What is it that economists are trying to understand exactly?
We want to predict how bidders are going to behave. Let’s suppose that I’m one of three bidders in one of these auctions, and I’m pretty sure that there’s going to be one bidder who will bid super high and another bidder who will bid very low. Probably my best move is to bid some number in the middle. If I wanted to win that first position, I’d have to outbid this really high bidder, which would be expensive. And I may as well outbid the low bidder because I can do so for cheap. So I’m going to bid some number in the middle. But then the question is: Which number in the middle?
It’s a very important question for the auctioneer, like Google, because my bid is going to set the price that the highest bidder pays, which will largely dictate the revenue of the auction.
KI: How does your refinement help answer this question?
If neither opponent trembles, then I’m indifferent among all these different bid amounts in the middle. But what if one of these other bidders trembles? What if one of them bids not exactly as I expect them to bid, even with just some small probability? If that is possible, then I might no longer be indifferent among all of these bids. Which of those bids I would prefer will depend on the trembles I expect. Is it more likely that the high bidder trembles or that the low bidder trembles—and if they do tremble, what alternative bids are they most likely to tremble to?
So there are these across-bidder comparisons that I need to make. Our refinement speaks to those comparisons and is going to help pin down a narrower range of possible bids that I, as this middle bidder, might make.
KI: How does this narrower range, the sharper prediction, benefit a search engine like Google?
There are other auction formats in the economics literature that in principle could also be used here. Then a relevant question for Google would be: Where is revenue going to be highest—if it sticks with this auction format that it’s using, or if it switches to a different auction format?
In order to do that comparison, Google would need a way to predict how a given set of bidders would bid in the generalized second-price auction and also how they would bid in another format. Our theory helps with the first half of that.
KI: Your coauthor, Paul Milgrom, just won the Nobel for his work on auction theory. He was originally your thesis advisor. How did you start working together?
I first saw him when I was a student in one of his classes. I was very impressed by the way he thinks, the way he explains things so clearly, and his contagious enthusiasm for, to be honest, for really nerdy stuff.
I started working for him as a research assistant. Then we started working on some projects together, and ultimately, he became my advisor.
KI: What has it been like recently to see auction theory in the news?
I’m really excited about it, and I’m very happy for Paul. What I think is really cool about Paul’s work and also that of Bob Wilson, his cowinner, is that it’s this brilliant theory, but it’s also incredibly practical. They’ve both been involved in designing auctions that have been used to allocate billions of dollars of radio spectrum. I think that mixture of brilliant theory and practical relevance is super rare and super special.