Innovation Jul 8, 2019
Artificial Intelligence Is Improving How Companies Do Business
Here are four of the most significant trends.
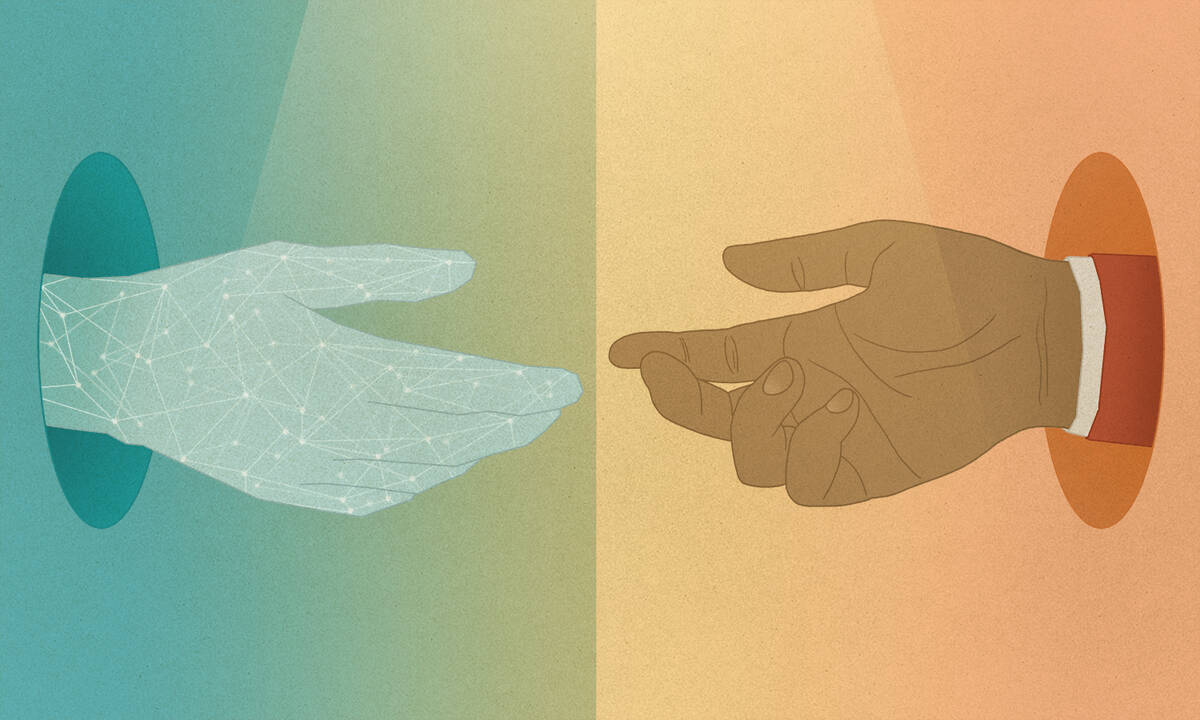
Lisa Röper
From savvier chat bots to automated financial planners, every day seems to bring news of yet another way artificial intelligence is changing how businesses find and serve their customers. But what’s the hype, what’s happening, and where is all this headed?
A version of this article was originally published in November of 2017. It has been updated to reflect current trends.
Adam Pah is a clinical associate professor of management and organizations at Kellogg, as well as a researcher at the Northwestern Institute on Complex Systems (NICO). Over the years, Pah has collaborated with a number of organizations as they integrate AI into their operations.
Here he highlights some of the most significant trends in AI that are changing how companies market to customers.
Using Natural Language and Video to Glean Customer Insights
People communicate mostly through natural language—not equations or Excel spreadsheets or structured daily reports. This has historically posed a challenge for marketers, because while the words we use are full of consumer insights, they have been generally inscrutable to analytical tools.
This is, of course, changing. With natural-language processing and machine-learning software, companies can now scrape vast libraries of text to generate insights on almost any subject. It has now become commonplace for companies to analyze, say, customers’ Facebook comments to design more effective digital-marketing strategies.
But organizations are becoming more creative and ambitious in thinking about how to apply insights from unstructured data, according to Pah.
Consider a cell-phone manufacturer launching a new phone.
Because these manufacturers make most of their sales through service providers such as ATT or T-Mobile, they are not directly connected to their customers. This means that post-launch customer feedback has historically been pulled from either customer returns or the manual monitoring of social media and tech websites by a small group of employees.
Pah worked with a manufacturer to create a different process. “We wrote a program that crawled the Internet for mentions on Twitter and Facebook along with about 30 sites where people review and talk about cell phones,” he says. “We then built a machine-learning solution that would automatically identify when the company’s product was being talked about, which features of the phone were being talked about, whether they were being talked about as positive or negative attributes. Then the program would group like statements together to give an idea about the magnitude of each problem—and it did this continuously.”
This new software allowed the phone manufacturer to start responding to issues immediately upon launch instead of after the typical six-week lag. It also provided a wealth of consumer insights that could be translated into future research and development.
Similar machine-learning efforts are well underway for images and video as well. There is a company, for instance, that monitors social-media feeds, televised sporting events, and shopping sites to identify when a logo appears in an image, video, or GIF. Another company analyzes and tracks the features of clothing popular with customers to determine which are trending. They then turn these insights into new styles, debuting over 1,000 new styles per week.
“You can now expand your focus beyond what people write and track Instagram as a way to understand when and where you are showing up, as well as whom you should be targeting,” says Pah.
“Typically, there is a trade-off between the usefulness of an AI tool and the amount of privacy a user enjoys.”
Making Smart Recommendations
Making recommendations is one of AI’s “oldest friends,” Pah says. Consider Amazon and Netflix matching customers’ past behavior with other products or movies they might like.
Pah says the push to make more accurate recommendations is showing no signs of slowing—and here is another area where advances in natural-language processing and image recognition are pushing the industry forward.
Olay, for instance, has an app that allows customers to upload a photo of their own face so that its algorithm can suggest relevant skincare products. That’s a key advance, he says, given that most recommendation engines are still based largely on what the consumer has previously bought. This can be a problem if customers have never before purchased a product in that category, are interested in truly novel products, or haven’t been happy with their earlier purchases.
For instance, when it comes to skincare products, “I generally get whatever is on sale,” Pah says. “So my past purchases are awful if you’re trying to help me actually take care of my skin.”
Pah points out that the art of using image recognition to generate recommendations is still being perfected: Olay’s app still requires users to manually provide the app with a lot of information about their skin, for example. “We’re not entirely there yet,” he says.
But the idea is right. “It’s actually pretty neat that they’re even trying.”
Privacy Concerns Are Growing. Companies Are Responding.
Another step in providing more useful recommendations involves incorporating data from a wider range of sources than we have seen historically. “So far, we haven’t moved much beyond this platform-centric world: some website recommends something else on the same website,” Pah says.
There are some signs this is changing. A few years ago, for instance, Pah received an email from TurboTax asking whether he would be interested in a machine-learning product that reviewed his tax returns, credit reports, and bank-account information to provide him with customized financial-planning advice.
“That’s a step in the right direction,” he says. “We’re starting to recognize that we need to bring in data from everywhere to make more specific and useful recommendations.”
But this would ultimately require an increase in cross-platform data sharing, a prospect that many—including customers, regulators, and even the platforms themselves—are starting to reconsider.
“Typically, there is a trade-off between the usefulness of an AI tool and the amount of privacy a user enjoys,” says Pah. In the past few years, “the data have become more siloed. Some of that is in response to the European Union; a lot more of that is in response to sifting through the stones of the past and realizing that companies made a lot of errors in how they handled private information.”
“Facebook still knows everything about you. Google still knows everything about you. But it would be much harder to start today, acquire the same amount of information about people as those players, and justify that collection.”
In response to restrictions on data sharing, Pah sees some companies turning to creative solutions—like synthetic data. Some large retailers, for instance, are using the customer data they do have to create millions of “unbelievably realistic” synthetic customers, Pah explains. These customer profiles are then fed into other deep-learning algorithms that learn how likely they are to respond to various marketing efforts. This additional data give the retailers the scale they need to improve their algorithms.
Take a straightforward example, such as knowing which coupons to send to which customers, when, and in which format. Instead of collecting a lot of information about a given customer from a range of sources, the retailer might generate numerous synthetic customers from the bits of information they do have and play out a number of scenarios to determine which coupon is most effective.
Synthetic customers still require some real data to generate, however. While long-standing companies like Sears—which have decades of data from loyalty programs and customer credit cards—are well positioned to build composite shoppers, smaller players might struggle.
But companies that can figure it out stand to reap huge benefits. “You have unlimited and infinite customers upon which to test,” Pah explains. “That, I think is, brilliant.”
Understanding that Data Is the Product
The increasing value of data is also encouraging startups to consider whether their products can do double duty: fill an immediate need for customers, but also create datasets that can be licensed to more established companies.
“People are really realizing the importance of data as a product,” says Pah.
Take Netradyne, a startup that uses AI to monitor whether drivers of large commercial fleets are operating safely. Its image recognition capabilities allow it to analyze dashcam video in real time, providing fleet managers with driving notifications.
That monitoring and analysis is the core product: “It can see if you’re driving through a stop sign; it can see if you’re driving too close to a car; it can see if you’re falling asleep,” explains Pah.
“The ancillary product is that they’re constantly mapping the roads, so they have road-map data,” he continues. These data could be useful for fleet managers aiming to make routes more efficient. Or they could help companies developing self-driving vehicles. “They’re in partnership talks with a number of automotive companies.”
It’s only a matter of time before marketers jump on this trend—if they haven’t already, says Pah.