Data Analytics Nov 5, 2021
4 Phases of Analytics Evolution: From Spreadsheets to AI Workbenches
What lies ahead for business leaders looking to incorporate data analytics?
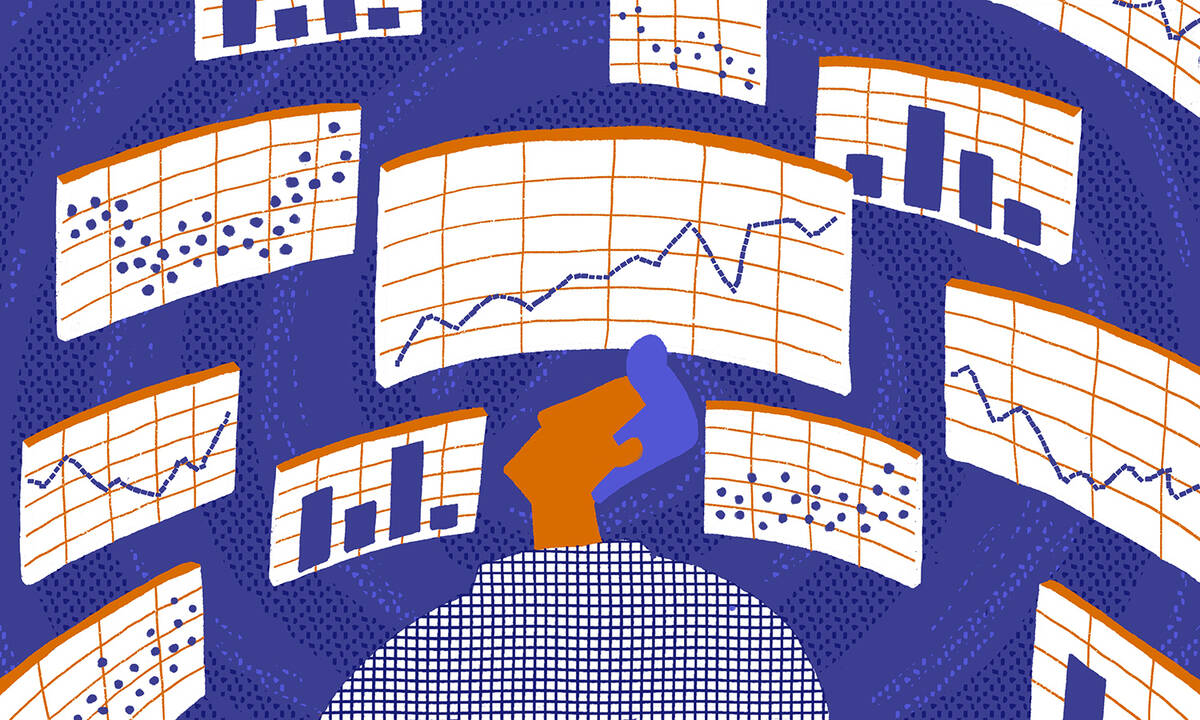
Riley Mann
Many years ago, I went looking for some “desktop AI”—artificial intelligence that would allow me to tease out trends in data. I knew about IBM’s Watson, so I went to the website in hopes of uploading data and getting access to some machine learning. “That’s not how it works,” a friend who worked at IBM told me at the time. “You can’t just press a button and get AI.”
That’s not the case anymore. Today, AI tools and workbenches allow you to click your way to some pretty advanced modeling techniques, without requiring any knowledge of coding or programming language. That’s certainly not to imply that everyone can be a data scientist. But business professionals today can have AI at their fingertips for making data-based projections. Sometimes, it’s as simple as clicking a button that says “predictor.”
To put this in perspective, let’s examine the four phases of data-analytics technology for the businessperson—three that brought us to this point, and one on what lies ahead.
Phase One—Simple Data Management
For years, businesses have been organizing information on spreadsheets—most notably Excel—to run analyses, such as summary statistics by group. Of course, to get value out of the tool, the user needed to understand the output; otherwise, the exercise was meaningless—and potentially dangerous when making decisions based on something not fully known or understood.
For example, back in the spreadsheet days, a data analyst might have compared customer purchasing behaviors across different groups or segments. Let’s say the analysis showed higher average purchasing among Segment 1 than Segment 2, which led to the conclusion that Segment 1 customers were more important to the business. A slightly deeper analysis of variability might reveal that Segment 2 customers were actually more consistent purchasers. That is, a better understanding of what the tool could produce yielded a more effective use of the tool.
While the spreadsheet was a great start for the everyday business professional’s analytic needs, it could be a blunt tool without the necessary nuances for deeper insights.
Phase Two—Powerful, but Inaccessible, Tools
The next phase brought advanced AI technology for analyzing data into the business-world mainstream. But until a few years ago, the most powerful AI analysis was limited to only the programmers and developers who built models with code. If someone wanted to compare 10 different models to see which one was most effective at predicting an outcome, it would be necessary to write code for 10 different models.
Phase 3—Powerful, Accessible AI Workbenches
Today’s new consumer-facing tech tools combine powerful algorithms with great user interfaces. Unlike the Phase 2 tools, AI workbenches no longer require knowledge of coding to build many AI models. Additionally, the best AI workbenches easily run many models simultaneously and identify the best model for the data.
What remains unchanged from the earliest days of simple data management, however, is the importance of understanding the outcome. When an algorithm produces a result, there are right and wrong, simple and nuanced, ways of using that result.
Business professionals today can have AI at their fingertips for making data-based projections.
— Joel Shapiro
Returning to the example of the customer segments, if an AI model indicates that a certain customer segment is likely to increase in value, humans still need to decide what to do about it. Should they engage more with these customers to ensure a good outcome occurs or keep the status quo because these customers are likely to lead to a good outcome on their own? In other words, it takes human expertise, and AI provides valuable inputs to supplement that expertise.
I recently saw this in action as I watched a business team making decisions of how to deploy sales-team talent and resources in the coming year. Without any formal data-science training, the team had built an AI-generated graphic that predicted with high accuracy the purchasing behaviors of AI-defined customer segments.
Five years ago, this same team would have needed an expensive external data-analytics resource to tackle this project. But more likely, they would never have thought about building a predictive model in the first place. Today, though, this analysis is part of how they do business.
A recent Harvard Business Review article stated that the digital transformation that has emerged from the Covid pandemic has shown the importance of data analytics and AI for business leaders. It cited a recent survey by The AI Journal that found 72 percent of business leaders believe AI will play a positive role in the future.
The question, then, is what the future of consumer-facing AI tools will look like.
Phase 4—The Next Frontier of Analytics Tech
As we look ahead, a new frontier of productivity is emerging as analytics insights are integrated into everyday business processes and workflows. Currently, common “desktop AI” tools can show how various factors relate to outcomes; therefore, it would not be a heavy computational lift to take the next step and use those relationships to prescribe some optimal mix of inputs. To be clear, I don’t just want to see what’s behind the models (that is, “glass box AI”); I want the model to show me the optimal mix of inputs for all potential outcomes, and to tie that to the benefits of being right and the costs of being wrong for my specific decision.
My wish list for the future would also include improvements in the integration of data. Increasingly, AI is getting better with relevant external data. This seems to indicate a future market boom around AI’s ability to identify valuable external datasets, and easily integrate and configure them.
From there, it’s natural to imagine a suite of decision-making tools that can be truly customized, putting the power of AI tools at business professionals’ fingertips. This won’t turn them into data scientists, nor will it dilute the value of professional data scientists. However, more seamless integration of AI into everyday business will increase its value and usability, provided that the outcomes are well understood.
*
This article originally appeared in Forbes.