Data Analytics Apr 1, 2019
To Improve Customer Experience, Embrace the Outliers in Your Data
Don’t ignore them. Let them highlight your biggest failures and juiciest opportunities.
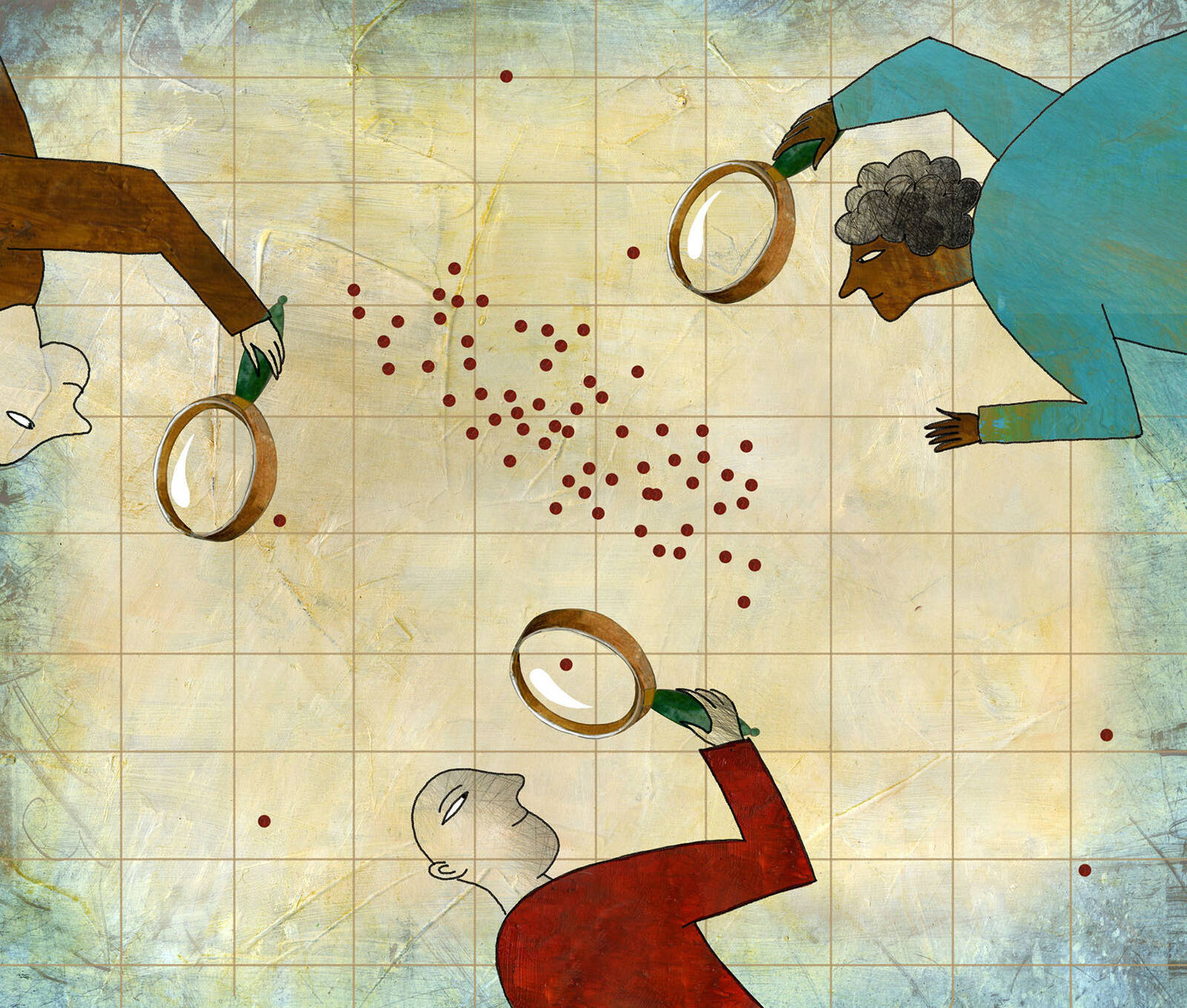
Yevgenia Nayberg
Companies are increasingly turning to data science to better understand how customers interact with their products and services. And with good reason. A 2018 survey sponsored by SAS, Accenture, and Intel found that 58 percent of responding business leaders said that using customer analytics significantly increased customer retention and loyalty, and nearly half associated analytics with significant revenue growth.
“It’s important to know if you’re providing the experience you want customers to have and making it better,” says Joel K. Shapiro, a clinical associate professor of data analytics at Kellogg. “To do that, you need to measure as much as you can about what happens to them.”
But according to Shapiro, far too many companies are ignoring some of the juiciest data around. These are the data that induce head-scratching, the measurements that don’t quite fit the existing models. These are the outliers. And they can highlight your product’s or service’s greatest weaknesses—as well as where it has the potential to truly shine.
Companies can and should use this knowledge to optimize the customer experience.
“The mere presence of outliers in customer experience data means that really good or bad things can happen to customers,” says Shapiro. “Maybe you can move that [experience] toward something that either increases the number of positive experiences or doesn’t detract from them.”
Keep Your Outliers
When data scientists come across an outlier, their first inclination may be to discard it in favor of “cleaning” or “smoothing out” the data. After all, the data might have been entered incorrectly or appear as the result of a modeling error. Or it may represent a freak accident—a set of circumstances unlikely to replicate itself. Why waste time accounting for the easily discountable?
Resist that urge, Shapiro says. It is always worth examining why the outlier occurred.
Shapiro comes to this insight firsthand. While studying the effectiveness of Spanish language instruction in West Virginia’s middle schools, he found that overall, students who received online instruction scored about the same as those who received it in person. But he also found that face-to-face class instruction produced a wider range of experiences. Investigating further, Shapiro learned that most of the high-performing in-person students came from one specific class.
After he had presented his results, the state’s board of education advised him to exclude that class from analysis because its instructor was known to work well with students. “They said she was the best thing that’s ever happened to the state of West Virginia from an education perspective,” Shapiro says.
But Shapiro realized that, rather than excluding this instructor’s students, the board of education should do the exact opposite. They should find out what this person was doing and try to replicate it.
What this outlier ultimately demonstrated was that, under the right circumstances, the in-person classroom model had the potential to outperform online instruction. This is an important first step toward understanding the potential of these outliers, Shapiro says. “They shouldn’t just be treated analytically and sort of smoothed over for some sort of aggregate analysis. They should be brought to the forefront.”
Get Some Context
It is important to note that Shapiro only learned of the exceptional teacher after disclosing his results to the state’s board of education. This highlights another critical step in utilizing data: business leaders must work closely with data scientists to interpret these outliers, as often the leaders are the only ones with the necessary institutional knowledge or business context.
“[Outliers] shouldn’t just be treated analytically and sort of smoothed over for some sort of aggregate analysis. They should be brought to the forefront.”
“It’s unfortunate when the business person, who is relying on the analysis, gets these aggregated trends without having those outliers identified to him or her by the data scientist,” Shapiro says. “The interaction with the data folks is critical so that the business experts can determine what’s really going on.”
Use Outliers to Optimize Automation
The Spanish instruction example hints at yet another important lesson about outliers: one of the biggest opportunities comes in knowing which aspects of the customer experience are ripe for automation, and which are best kept in-person.
Automation can produce a more consistent customer experience—that is, fewer outliers. Depending on the circumstance, this can be a good thing or a bad thing.
“Automation tends to create a ceiling on the best experience you can have and a floor on the worst experience,” Shapiro says. “It drives everybody to the middle.”
Think of it this way: If your cell phone breaks and you need to call your service provider, would you rather wait several minutes to speak with a friendly representative or have immediate contact with a chatbot—an artificial intelligence program that can answer basic inquiries quickly?
“Today’s chatbots may be able to solve my problem, but they probably won’t make me feel great about the experience,” says Shapiro. “If I’m talking to a live person, they might say ‘Hey, you’re in Chicago today. My goodness, I hear it’s -3 and the wind chill is -40. Is everyone okay?’ A human touch can provide an emotional connection that most automation can’t rival.”
Outliers can be useful for helping companies determine where a given customer interaction should fall along the continuum between in-person and automated, says Shapiro. “Every organization needs to answer for itself where it wants to inject variation and where it wants to standardize or automate that variation away.”
In other words, where do the benefits of an in-person interaction have the potential to outweigh the costs?
Know Your Brand
Shapiro recommends companies determine how each model of customer experience fits into its brand identity. For example, Oregon-based Dutch Bros. Coffee, known for its “bro-istas”—men and women who memorize customer preferences and dole out free coffee to down-on-their-luck patrons, leans toward personalization. Domino’s, on the other hand, has reemerged as a U.S. pizza market leader by automating customer orders through its mobile app.
“Automation, done well, can be highly efficient. Most places would say that when they fulfill orders, they’re okay with standardizing or automating to be quick and accurate,” Shapiro says. “But there are other elements of that customer journey that might merit a lack of automation.”
It also depends on a company’s desire to give up consistency in favor of a personal touch. Shapiro appreciates that many chain hotels now offer automated check-in service. But he also understands why others would want a front-desk clerk handling that job. “It could be important for them to establish a relationship,” he says. “For example, me chatting with the nice Midwesterner behind the counter of the Hilton hotel where I’m staying might be a really important part of their brand.”
That personal touch comes at a cost in staffing, of course. And, if those people are not trained properly and coached regularly, they can veer from established protocols or otherwise provide poor customer experiences. Companies with brands known for exceptional service might forego automation, but in their quest for that personal touch, they will need to invest in minimizing bad outcomes.
“The outliers indicate what your problems are and where your greatest successes are,” Shapiro says, “and they allow you to facilitate those great successes and eliminate the problems.”